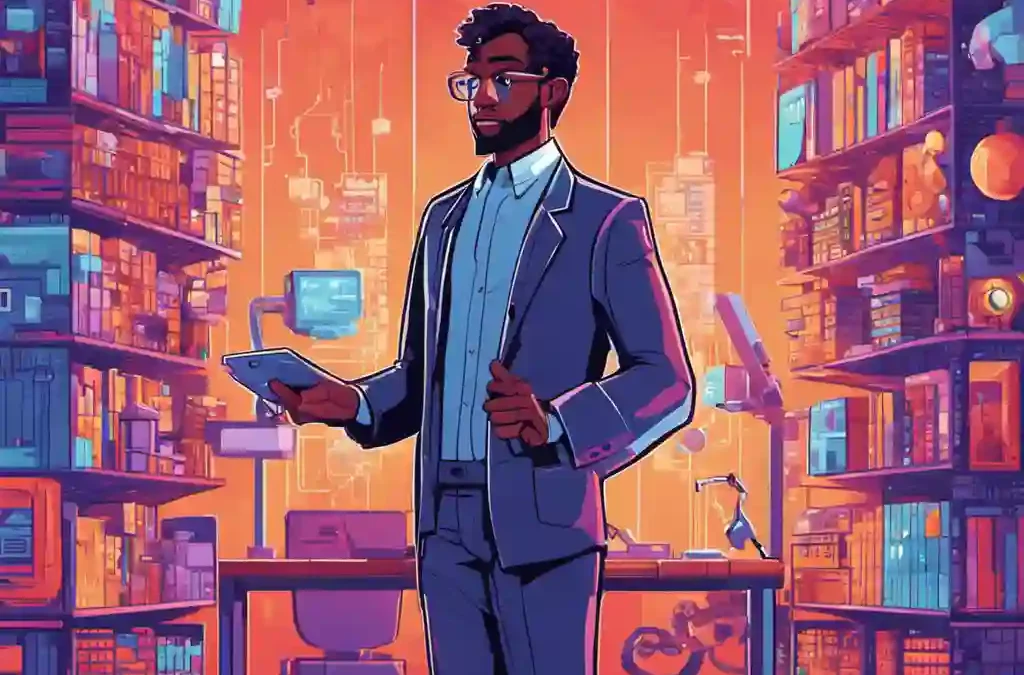
True Raw Power in Data Visualization in Data Science, Beyond AI, Beyond LLMs
Are you seeking to learn more about the importance of data visualization in data science and trying to get beyond what ChatGPT is saying? Explained by advanced analytics consultants who spend their time in Big Data Technology and have a rich history in Data Mining for enterprise companies?
You’re in luck—we are a legit consultancy based in Austin, Texas. We are originally a data visualization consulting services business that prides itself on its upbringing focused primarily on tableau consulting services. We work directly with experts on a weekly to monthly basis and have many valuable insights that we can share from our experiences, research, and time in production environments.
For those breaking into the data industry, welcome to your future!
What is data visualization?
At some point, you must answer this question: what is data visualization? Data visualization is drag-and-drop analytics for most people who can’t write SQL. Chart creation. However, for technical analytics gurus, we understand data visualization is an end-to-end stack of engineering, design, architecture, and total comprehension of requirements to be visualized.
An easy phrase is “see and understand data,” coined by Tableau in 2014, from what I recall. This was a good way to explain data visuals to non-technical audiences.
Data visualization, including exploratory graphics using tools like Tableau, is crucial to data science.
Please take a peek at our Tableau VS Power BI key differences and Data Governance Comprehensive Guide!
Visualizing data in this field often uses line and bar charts. These charts help us understand and interpret complex data and identify patterns, trends, and outliers.
Many graphics, such as those created using Tableau, can significantly enhance our understanding of the data and enable us to apply machine-learning techniques more effectively. This allows us to make informed decisions based on data insights using visualization tools in our reports, like Google Charts (for developers) and Tableau (for non-developers).
DEV3LOPCOM, LLC offers two types of data visualization consulting services for the past 10 years
One that is easy to create and support and one that requires a full-stack engineer to build and a very technical analyst to support. Many of our clients opt for the easy-to-build visualizations because they are easier for their analysts to adopt. This is the difference between prototyping and hardening. This can be saved for another blog.
Data visualization is a powerful tool that can change lives. I know this because data visualization has changed our lives.
Data visualization will enhance your future, improve your communication skills, and definitely change your ability to storytell with data. It helps us understand data, engage our audience, and share information more effectively. Using graphics and charts, we can create visually appealing presentations that clearly and compellingly convey our findings. Effective data visualization is essential for anyone looking to tell a data-driven story and make an impact with their conclusions.
By embracing the importance of graphics and data visualization in data science, we unlock new possibilities for understanding complex information, making informed decisions, and communicating insights effectively through data visualization.
Definition and Examples of Data Visualization
Data visualization is a powerful tool that can change lives. It transforms raw data into visual formats such as charts, graphs, maps, infographics, dashboards, and other visualization tools. This makes it easier to understand complex data by visually representing it through graphics and plots.
We mostly cover data visualization using applications like Tableau Software, Microsoft PowerBI, Qlik, and Google Data Studio (aka Looker).
Examples of Data Visualization Today
When discussing data visualization, we refer to various graphics and plot visual representations that help us make sense of the information. Here are some examples:
- Bar charts, line charts, pie charts, and scatter plots are commonly used graphics to display numerical data visually. Data visualization processes allow us to generate presentations with a graph or more to tell a data story and easily compare different categories or trends in data analysis. With the help of data visualization tools, we can effectively visualize and analyze big data, making it easier to understand and draw insights from.
- Data visualization methods such as a network graph, heat map, and tree diagram are helpful when showing relationships between entities or hierarchical structures. These tools are essential in data science visualization, allowing for clear and concise representation of complex information. Graphics play a crucial role in conveying the data effectively. Graphics and plots help us understand connections and dependencies within a dataset.
- Geographic maps are powerful tools for displaying location-based data, especially regarding graphics and plots. Data science visualization can show population density, weather patterns, or even the spread of diseases across regions through graphics and plots, creating a compelling data story.
- Infographics, a form of data science visualization or exploratory graphics, combine text and visuals to concisely and engagingly convey complex information. In data science visualization, graphics are often used to enhance understanding. They often use icons, illustrations, and color schemes to enhance understanding.
- Dashboards are essential for data science visualization, testing a data visualization tool, creating a data story, and helping data graphs as they offer a real-time overview of key metrics or performance indicators. They bring together multiple graphics on a single screen for quick analysis.
The Importance of Data Visualization
Now that we have explored some examples of data visualization let’s discuss why graphics are essential in data science.
- Visual representations, such as graphics, let you plot comprehension by simplifying complex datasets and presenting them in a more digestible format. Instead of sifting through rows, stressing about the data process, and digging through columns of numbers, we can quickly grasp patterns and trends, use a visualization tool, and share outliers at a glance with data science visualization and graphics.
- Efficient Analysis: With large volumes of graphics data becoming increasingly common today, analyzing raw numbers alone can be overwhelming and time-consuming. Data visualization allows analysts to explore and gain insights from vast datasets more efficiently using graphics.
- Enhanced Decision Making: When graphics present data visually, it becomes easier to identify correlations, spot anomalies, and draw meaningful conclusions. This empowers decision-makers to make informed choices based on a comprehensive understanding of the graphics data.
- Improved Communication: Data science visualization graphics have a universal language that transcends barriers and lets us tell a data story through a presentation layer, software. Using data visualization software, we can effectively communicate complex ideas or findings to diverse audiences, regardless of their technical expertise. Graphics play a crucial role in conveying information visually.
- Data visualization enables us to tell compelling stories with our graphics and also helps us work on our data process. Storytelling with data is a powerful way to engage, plot value, and communicate information effectively. By carefully selecting suitable graphics and arranging them logically, we can guide our audience through a narrative highlighting key insights and takeaways.
- Collaboration and Sharing: Graphics foster collaboration among team members by providing a common framework for discussing and analyzing data visualizations. They also facilitate sharing graphic findings with stakeholders who may not be directly involved in the analysis process.
- Identifying Patterns and Trends: Visual representations in a visualization tool, such as graphics, help us identify patterns, trends, and relationships that might go unnoticed in raw data. These insights can then be used to predict or inform future graphics strategies.
- Real-Time Monitoring: Dashboards with live graphics allow organizations to monitor metrics in real-time. This helps them stay agile and respond promptly to graphics changes or anomalies as they occur.
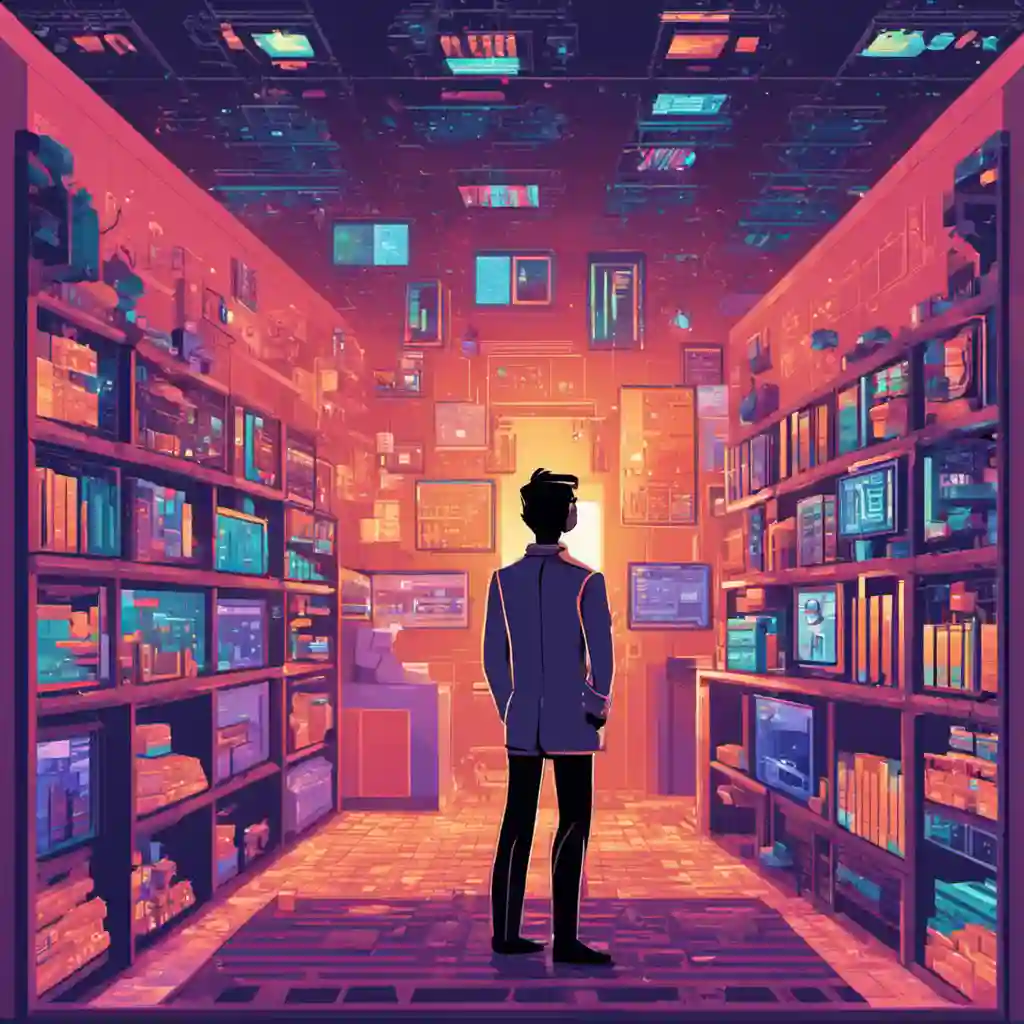
Benefits of Data Visualization in Data Science
Data visualization is crucial in data science projects, offering several benefits in understanding complex concepts and findings through graphics. Explore some key advantages of incorporating graphics and data visualization into data science workflows.
Simplifying Complex Concepts and Findings
Data science involves working with vast amounts of information and analyzing it to extract meaningful insights, including graphics. However, raw data can be overwhelming and challenging to comprehend without graphics. This is where data visualization comes in handy. Presenting data visually through graphics, such as charts, graphs, and other visual representations, simplifies complex concepts and makes them more accessible to everyone.
Identifying Correlations, Causations, and Relationships
One of the primary goals of data analysis is to identify relationships between variables or factors while using our emerging technology data visualization tool. Data visualization, with the help of graphics, allows us to quickly identify correlations, causations, and patterns within datasets. Visualizing these graphics relationships makes it easier for data scientists to draw conclusions and make informed decisions based on their findings.
What is Correlation?
Correlation refers to the statistical relationship between two variables. It measures how closely one variable changes when another variable changes. In other words, correlation measures the strength and direction of the linear relationship between two variables. There are several types of correlations: positive, negative, and no. A positive correlation means that the other variable also tends to increase as one variable increases. A negative correlation means that the other variable decreases as one variable increases. No correlation means that there is no linear relationship between the two variables.
What is Causation?
Causation refers to the cause-and-effect relationship between two or more variables. It states that one variable (the cause) can influence another variable (the effect). In other words, causation describes how one variable affects another variable. There are several types of causation, including direct and indirect causation. Direct causation means that the cause directly influences the effect. Indirect causation means the cause indirectly controls the impact through a third variable or factor.
What is a Relationship?
Relationships, like SQL Joins, refer to the connection between two or more variables. It can be either correlative, causal, or both. A correlative relationship means a statistical relationship between two variables, like the Pearson Correlation. A causal relationship implies that one variable causes another variable. Correlative and causal relationships are essential in data analysis because they help us understand how variables interact.
Master SQL syntax with us in our comprehensive article.
Spotting Anomalies or Errors
In any dataset, anomalies or errors in graphics may need to be identified and addressed when hunting through our data visualization tool. These graphics outliers can significantly impact the accuracy of the analysis if not detected early on. Data visualization enables us to visualize the distribution of data points effectively, which is highly beneficial when you dive into machine learning, making it easier to spot anomalies or inconsistencies within the dataset. Graphics play a crucial role in this process.
Imagine learning machine learning without the ability to spot an anomaly yourself. It often takes many graphics to plot the correct line and stories; however, it’s a variable game of checking, testing, fixing, and continuously supporting over time.
Learn to spot anomalies using Market Basket Analysis in our comprehensive article.
Presenting Results Effectively
Data scientists often must present their findings to stakeholders or non-experts who may not have a technical background and the data sets they know because they are often SMEs (subject matter experts). To effectively communicate their results, data scientists can utilize graphics to represent complex information visually, and usually, many graphics are required to turn heads.
In such cases, compelling graphics become essential for conveying complex information clearly and concisely, like showing the distribution between two vertical bars, which may be needed for a big data visualization solution in the future!
Graphics are visual representations that make it easier for others to understand the insights from the analysis without getting overwhelmed by numbers, technical jargon, or the many graphics that do not make sense.
To summarize:
- Data visualization simplifies complex concepts and data science project findings using graphics.
- Graphics facilitate the identification of correlations, causations, and relationships within datasets, and they provide visual representations that make it easier to analyze and understand data.
- Graphics, or visual representations, help identify anomalies or errors in the collected data.
- Effective graphics aid in presenting results to stakeholders or non-experts.
Importance of Data Visualization Software in Business Analytics
Data visualization plays a vital role in business analytics by providing actionable insights for decision-making processes. Graphics are essential in effectively communicating complex data to stakeholders. Businesses can easily interpret and understand complex data by transforming it into visual representations like graphics. Let’s explore why data visualization, mainly graphics reports, is essential in business analytics.
Visualizing business metrics for monitoring performance
One of the key benefits of data visualization software in business analytics is its ability to help monitor performance against key objectives, especially when it comes to graphics. Instead of relying on raw numbers and spreadsheets, visualizations allow businesses to track their progress visually using graphics. With clear and intuitive graphics, graphs, charts, and dashboards, it becomes easier to identify trends, patterns, and anomalies within the data.
For example, suppose a retail company wants to analyze its sales performance across different regions using graphics. By visualizing this data using graphics such as a map or a bar chart, they can quickly identify which areas are performing well and which need improvement. This enables them to make informed decisions about resource allocation, marketing strategies, and graphics.
Real-time tracking with interactive dashboards
Interactive dashboards are another powerful tool provided by graphics in business analytics. These dashboards allow businesses to track Key Performance Indicators (KPIs) in real-time. Users can drill down into specific metrics with just a few clicks or taps and gain deeper insights into their performance.
Imagine you’re managing an e-commerce website and want to monitor your website traffic. An interactive dashboard could display real-time metrics such as page views, bounce, and conversion rates. We created Colibri Google Analytics Tableau Dashboard to help us view multiple domains. By dynamizing this information, you can identify sudden changes or issues requiring immediate attention.
Effective communication of business strategies
Clear visualizations also play a vital role in communicating business strategies across teams within an organization. When presenting complex ideas or plans during meetings or presentations, visuals help convey information more effectively than lengthy reports or verbal explanations alone.
For instance, if you’re introducing a new marketing campaign to your team, a visually appealing infographic or chart can simplify the message and make it more memorable. Visuals help everyone understand the campaign’s goals, target audience, and expected outcomes.
Enhanced decision-making with data visualization
Data visualization empowers businesses to make informed decisions by visually representing complex data sets. Instead of relying on intuition or guesswork, decision-makers can analyze trends and patterns within the data to identify opportunities or potential risks.
Let’s say you’re a business owner trying to decide whether to expand into a new market. By visualizing relevant data such as population demographics, consumer behavior, and competitor analysis, you can gain insights into the market’s potential profitability and make an informed decision.
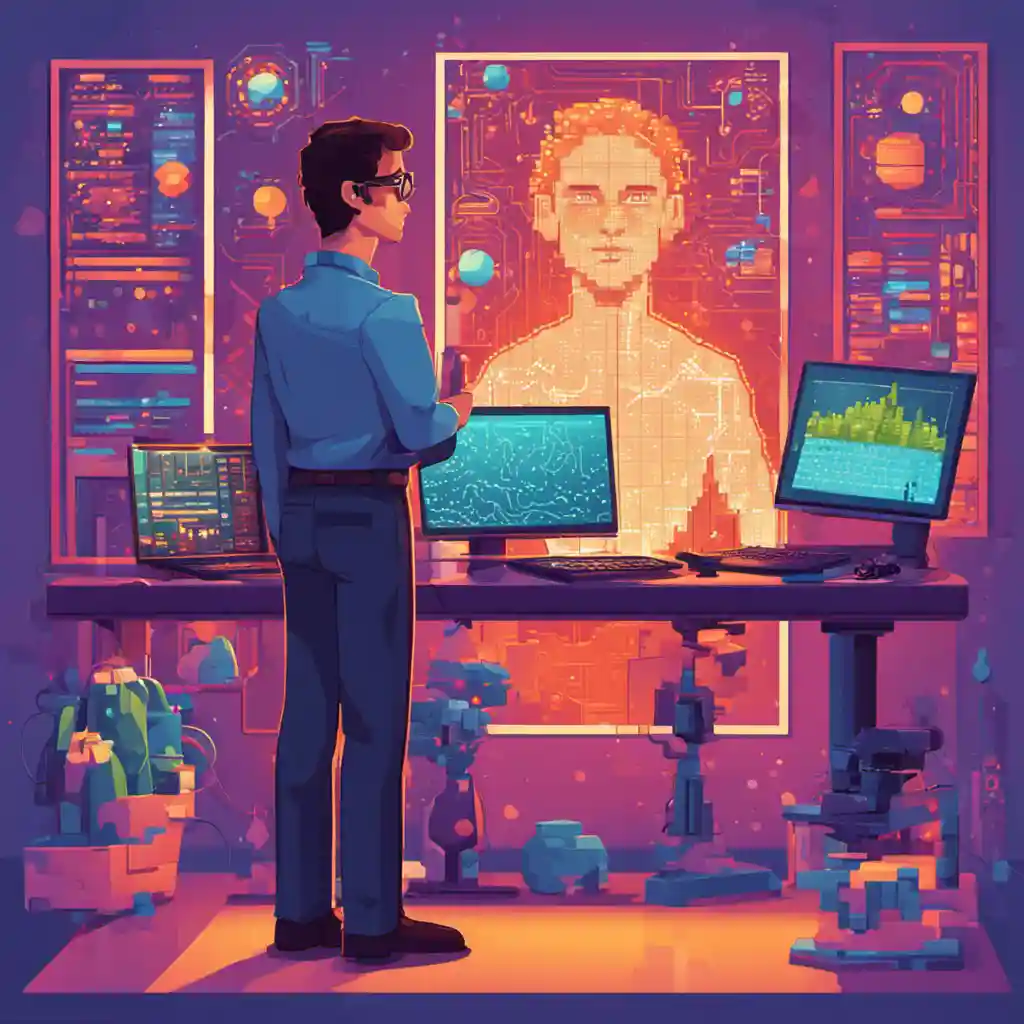
Significance of Data Visualization in Big Data Analytics
Data visualization plays a crucial role in the field of big data analytics. Effective visualization strategies are essential to handle large volumes of information. Let’s explore why data visualization is so significant in big data analytics.
Simplifying Analysis and Interpretation
Visual representations provide a simplified way to analyze and interpret information when dealing with massive datasets. Instead of sifting through endless rows and columns of raw data, visualizations present the data in a graphical format that is easy to understand. This allows analysts to identify dataset trends, patterns, and outliers quickly.
Interactive Visualizations for Easy Exploration
One of the key benefits of data visualization in big data analytics is its interactivity. Interactive visualizations allow users to quickly drill down into specific subsets or details within large datasets. Analysts can explore different dimensions and variables by interacting with the visualizations, gaining deeper insights into the underlying data. This level of exploration would be challenging without visual representations.
Uncovering Hidden Trends and Patterns
Data visualization enables businesses to uncover trends or patterns that may have otherwise been hidden within complex datasets. Visually representing the data makes it easier to spot correlations, anomalies, or emerging patterns that might not be apparent when looking at raw numbers alone. These insights can help organizations make informed decisions and exploit new opportunities.
Communicating Insights Effectively
In addition to simplifying analysis and interpretation for analysts, data visualization facilitates effective communication of insights across teams or stakeholders. Visual representations make it easier for non-technical individuals to grasp complex concepts by presenting information visually appealingly. This ensures everyone can understand and act upon the insights derived from big data analytics.
Enhancing Decision-Making Processes
By providing explicit visual representations of complex datasets, data visualization empowers decision-makers to make well-informed choices based on accurate information. When faced with large volumes of data, it can be challenging to identify the most relevant information or trends. Visualizations help decision-makers focus on the key insights and make data-driven decisions quickly and confidently.
Improving Data Quality and Accuracy
Data visualization also plays a role in improving data quality and accuracy within big data analytics. When visualizing data, inconsistencies or errors in the dataset become more apparent, allowing analysts to identify and rectify them promptly. Organizations can have confidence in their analytics results by ensuring the underlying data is accurate and reliable.
Enhancing Collaboration and Knowledge Sharing
Data visualization promotes collaboration and knowledge sharing among teams working on big data analytics projects. Visual representations make it easier for team members to share insights, discuss findings, and collaborate on problem-solving. This collaborative approach helps harness the collective intelligence, leading to more comprehensive analyses and better outcomes.
Role of Data Science Visualization: Tools and Techniques
Data science visualization plays a crucial role in analyzing and interpreting data. It involves using various tools and techniques to create meaningful visual representations that help us understand complex information more easily. Let’s explore the different tools, techniques, and benefits of data science visualization.
Data Visualization Tools
In data science, several powerful tools are available for creating visualizations. Some popular ones include Tableau, Power BI, and Python libraries such as Matplotlib and Seaborn. These tools provide a user-friendly interface with drag-and-drop functionality, making it easier for data scientists to create interactive dashboards and visualizations.
Data Visualization Techniques
Different types of data require other visualization techniques. Heatmaps represent patterns or correlations in large datasets, while scatter plots help visualize relationships between variables. Histograms help understand the distribution of numerical data, and network diagrams display relationships between entities.
Exploring Data and Identifying Outliers
Data scientists use data visualizations to explore datasets thoroughly. By creating visual representations of the data, they can identify patterns, trends, outliers, and anomalies that may not be apparent from raw numbers alone. For example, a scatter plot might reveal a strong positive or negative correlation between two variables, providing valuable insights into their relationship.
Validating Models
Visualization also plays a vital role in validating models in data science. Data scientists can effectively assess their models’ accuracy by comparing predicted outcomes with actual results through visualizations. This helps them identify any discrepancies or areas for improvement in their algorithms or methodologies.
Effective Communication with Stakeholders
One significant advantage of data visualization is its ability to present findings and insights to stakeholders effectively. Visual representations make complex information more accessible and understandable for non-technical audiences. Instead of giving them tables full of numbers or lengthy reports filled with jargon, visualizations clearly and concisely convey information.
Enhancing Data Analysis
Visualization tools enable data scientists to perform in-depth analysis of large datasets. With the ability to interact with visualizations, they can drill down into specific data points, apply filters, and uncover hidden insights. This interactivity allows for a more comprehensive understanding of the underlying data and helps identify trends, patterns, or anomalies that may have otherwise gone unnoticed.
Improving Decision-Making
Data visualization empowers organizations to make informed decisions based on data-driven insights. By presenting complex information visually, decision-makers can quickly grasp the essential findings and implications. Visualizations provide a holistic data view, enabling stakeholders to identify trends, outliers, and potential risks or opportunities that may influence strategic decisions.
Exploring Different Types of Data Visualization
Data visualization is a crucial aspect of data science. It helps us understand and interpret complex datasets by presenting them visually.
Bar Charts
Bar charts are one of the most common types of data visualizations. They use vertical bars to represent different categories or groups and their corresponding values. Bar charts are excellent for comparing quantities across various categories or tracking changes over time.
Line Graphs
Line graphs are ideal for showing trends and patterns over time. They use lines to connect data points, allowing us to visualize how variables change about one another. Line graphs are often used in analyzing stock market trends, weather patterns, and population growth.
Pie Charts
Pie charts display data as slices of a circle, with each slice representing a proportion or percentage of the whole. They help illustrate parts-to-whole relationships or compare relative sizes of different categories within a dataset.
Scatter Plots
Scatter plots are excellent for exploring relationships between two numerical variables. Each point on the plot represents an observation, with one variable plotted on the x-axis and the other on the y-axis. By examining the distribution of points, we can identify correlations or clusters within the dataset.
Treemaps
Treemaps provide a hierarchical view of data by dividing rectangles into smaller rectangles based on their proportions. Each rectangle represents a category or subgroup, with larger rectangles indicating higher values or frequencies. Treemaps help us analyze complex datasets that have multiple levels of categorization.
Heat Maps
Heat maps use colors to represent values within a matrix or grid-like structure. They are handy for visualizing large amounts of data and identifying patterns or anomalies quickly. Heat maps are commonly used in genetics, finance, and geographic information systems (GIS) fields.
When choosing the right visualization type, it’s essential to consider the purpose and characteristics of the dataset. Different visualizations offer unique perspectives on the same data, allowing us to uncover insights that may not be apparent through other methods.
For example, a bar chart would be an excellent choice to compare sales figures across different product categories. On the other hand, if we want to examine how two variables are related, a scatter plot would provide a more in-depth analysis.
The choice of colors is also crucial in data visualization. Colors can highlight specific patterns or draw attention to particular data points. However, it’s important not to overload the visualization with too many colors, as this can make it difficult for viewers to interpret the information accurately.
In addition to selecting the appropriate visualization type and color scheme, it’s essential to ensure that the visual representation is clear and easy to understand. Labels and titles should be used effectively to provide context and guide viewers through the information presented.
Data visualization is vital in data science by helping us explore and analyze complex datasets effectively. Using different types of visualizations, such as bar charts, line graphs, pie charts, scatter plots, treemaps, and heat maps, we can gain valuable insights into our data and communicate those findings clearly to others.
The Growing Importance of Data Visualization
Effective visualization becomes crucial for understanding trends and patterns as data grows exponentially. In today’s digital age, we generate massive amounts of data every second. From social media posts to online transactions, a constant influx of information needs to be analyzed and interpreted. This is where data visualization makes sense of the vast sea of numbers and figures.
Organizations increasingly rely on visualizations to gain a competitive edge in their industries. Raw data can often be overwhelming and challenging to comprehend. However, when presented visually, it becomes much easier to identify patterns, correlations, and outliers. Visualizations help us see the bigger picture and extract valuable insights from complex datasets.
With advancements in technology and tools, creating interactive visualizations has become more accessible. Gone are the days when only experts could create visually appealing graphs or charts. With user-friendly software like Google Charts and Tableau, anyone can transform raw data into captivating visuals without extensive coding knowledge.
Data visualization plays a massive role in storytelling with data. It allows us to present our findings in a compelling way that engages the audience and conveys information effectively. Using various graphic elements such as color coding, bar charts, heat maps, or line graphs, we can tell a story behind the numbers and communicate complex ideas.
The importance of data visualization cannot be overstated when dealing with big data. Visual representations provide clarity and facilitate decision-making processes when working with huge volumes of information that may seem incomprehensible at first glance. By condensing large datasets into easily digestible graphics or infographics, we can quickly identify trends or anomalies that might have gone unnoticed.
Visualizations also enable us to explore multiple dimensions simultaneously by representing different variables on a single graph or chart. This helps us uncover hidden relationships between variables that may not be apparent through traditional statistical analysis alone.
In addition to its practical applications, data visualization also has aesthetic value. Well-designed visualizations can be visually appealing and captivating, making it easier for the audience to engage with the presented information. By using colors, shapes, and other design elements effectively, we can create visually stunning representations that enhance understanding and retention of information.
The demand for professionals skilled in data visualization is rising across various sectors. Companies recognize the need for individuals who can analyze and present data visually compellingly. From marketing departments looking to understand consumer behavior to healthcare organizations seeking insights from patient records, there is a growing need for data visualization experts who can transform complex data into meaningful visuals.
Exploring Jupyter Notebook and Recommended Books on Data Visualization
Jupyter Notebook is an open-source web application that allows you to create interactive notebooks with code snippets and visualizations. It’s a powerful tool for data scientists and analysts to explore, analyze, and communicate their findings effectively.
Jupyter Notebook: A Versatile Tool for Data Scientists
Jupyter Notebook provides a flexible environment for working with data using various programming languages such as Python, R, Julia, and more. Its intuitive interface seamlessly combines code execution with text explanations, equations, images, and visualizations in a single document.
One of the critical benefits of the Jupyter Notebook is its ability to display visualizations directly within the notebook itself. By leveraging popular Python libraries like Matplotlib, Seaborn, Plotly, or Bokeh, you can create stunning charts, graphs, maps, and other visual representations of your data. This interactive nature allows you to explore your datasets dynamically and gain deeper insights.
Another advantage of using Jupyter Notebook is its collaborative features. Multiple users can simultaneously work on the same notebook by sharing it through platforms like GitHub or Google Colaboratory. This fosters teamwork and enables seamless knowledge sharing among data science teams.
Overlapping Use Cases and Types of Data Visualization
Data visualization plays a crucial role in uncovering insights and making informed decisions. Let’s explore some everyday use cases where data visualization comes into play and the different types of visualizations that are commonly used.
Sales Analysis
One critical use case for data visualization is sales analysis. Companies often need to analyze their sales performance across different regions or products. This is where bar charts come in handy. Bar charts provide a simple and effective way to compare sales performance visually. By representing each region or product as a separate bar, it becomes easy to identify trends, spot anomalies, and make data-driven decisions.
Customer Segmentation
Understanding customer behavior is essential for businesses to tailor their marketing strategies effectively. Heatmaps are a powerful tool for visualizing customer behavior patterns over time. Using color gradients to represent varying levels of engagement or activity, heatmaps allow businesses to identify hotspots or areas that require attention. This information can be invaluable.
Financial Forecasting
Financial forecasting involves predicting future economic outcomes based on historical data and market trends. Data visualization is crucial in providing an intuitive understanding of complex financial information. Line graphs commonly visualize historical trends, allowing analysts to identify patterns and accurately predict future financial performance.
Supply Chain Optimization
Efficient supply chain management is vital for businesses operating in various industries. Network diagrams are an excellent tool for understanding the intricate relationships within a supply chain network. These diagrams help visualize how different entities, such as suppliers, manufacturers, distributors, and retailers, are connected and interact. Businesses can optimize their supply chain operations and improve overall efficiency by identifying bottlenecks or inefficiencies within the network.
It’s essential to consider the nature of your data and the insights you want to uncover. Different types of visualizations excel in different scenarios, so let’s take a closer look at some commonly used ones.
Bar Charts
Bar charts are versatile and widely used for comparing categorical data. They are instrumental when comparing values across different categories or groups. For example, if you want to compare the sales performance of other product lines, a bar chart can provide a clear visual representation of how each product line is performing.
Heatmaps
Heatmaps are excellent for displaying large amounts of data in a compact and visually appealing format. They use color gradients to represent varying levels of intensity or value. Heatmaps are commonly used in various fields, such as customer analytics, website user behavior analysis, and risk assessment.
Network Diagrams
Network diagrams help visualize complex relationships within a network by representing entities as nodes and connections as edges. These diagrams are often used in social network analysis, supply chain management, and cybersecurity to understand connectivity patterns and identify key influencers or vulnerabilities within a network.
Everyday Use Cases for Data Visualization
Data visualization plays a crucial role in various fields, helping us make sense of complex information and uncover valuable insights. Let’s explore some everyday use cases where data visualization is widely used.
Data Exploration
One of the primary uses of data visualization is to explore and understand large datasets. Data scientists can quickly identify patterns, trends, and relationships within the data by creating visual representations such as charts, graphs, and maps. For example, we have a dataset containing information about customer purchases in an online store. Visualizing this data using line charts or scatter plots allows us to easily spot popular products, peak buying times, or correlations between price and customer satisfaction.
Trend Analysis
Another important application of data visualization is trend analysis. Visualizing historical data over time allows us to identify long-term patterns or changes in various metrics. This can be especially useful for businesses looking to analyze sales performance or track market trends. For instance, marketing teams can use line charts to visualize website traffic over several months to determine if their marketing campaigns drive more visitors.
Anomaly Detection
Data visualization also helps in detecting anomalies or outliers within datasets. Anomalies are observations that deviate significantly from the expected behavior or pattern. By visualizing the data using techniques like box plots or heatmaps, analysts can quickly spot these anomalies that may indicate errors in the dataset or highlight unusual events worthy of further investigation. For example, healthcare professionals can use visualizations to monitor patient vital signs and detect abnormal readings that may require immediate attention.
Example of detection in politics: In democratic societies, it’s essential that elections are fair and everyone’s vote counts the same. Elections are like extensive experiments where people vote based on what they want. We can see if any cheating is happening by looking at the results in a certain way. We found that the results differ from ordinary elections where there might be cheating. For example, we looked at Russian elections and found evidence of cheating with fake ballots. We made a model to measure how much cheating is happening. We also made a test to check for these cheating patterns in election results. The cool thing is that this method works well no matter how detailed the data is, so we can compare different countries.
Forecasting
Forecasting future trends and making predictions is another area where data visualization proves invaluable. Organizations can create visualizations that provide insights into future outcomes by analyzing historical data patterns and using statistical models. These forecasts help businesses make informed decisions regarding resource allocation, budget planning, inventory management, etc.
Now let’s explore some real-world examples of how data visualization is used in different industries:
- Healthcare: Visualizations are widely used in healthcare to monitor patient data, identify disease patterns, and track the spread of epidemics. For instance, visualizing geographic data on a map can help identify areas with high infection rates during a disease outbreak.
- Marketing: Marketing teams utilize data visualization to analyze campaign performance and customer behavior. By visualizing metrics like click-through rates, conversion rates, or customer segmentation, marketers can gain valuable insights into the effectiveness of their marketing strategies.
- Government: Government agencies employ data visualization with their machine learning and a bar chart, line chart, or heat map to understand demographic trends, user analysis, plot trends, create a data story, and make informed policy decisions. Visualizations can help policymakers identify areas with high crime rates or visualize population growth patterns to plan infrastructure development accordingly.
Conclusion: The Importance of Data Visualization in Data Science
In conclusion, data visualization is a crucial component of data science that cannot be overlooked. It goes beyond just presenting information in a visually appealing manner; it allows us to understand complex datasets and extract meaningful insights. By representing data through charts, graphs, and interactive visuals, we can uncover patterns, trends, and correlations that might otherwise remain hidden.
Data visualization not only aids in better decision-making but also enhances communication and storytelling. It enables us to convey our findings effectively to technical and non-technical audiences, making it an essential skill for any data scientist or analyst. So whether you’re exploring business analytics or diving into big data analytics, mastering the art of data visualization will undoubtedly elevate your work.
Now that you understand the importance of data visualization in data science, it’s time to put your knowledge into practice. Start exploring different tools and techniques available for creating impactful visualizations. Don’t shy away from experimenting with various types of visual representations to find the most suitable ones for your specific use cases. Remember, the true power of data lies not just in its collection but in how we transform it into actionable insights through compelling visual narratives.
FAQs
Why is data visualization important?
Data visualization is important because it helps us make sense of complex datasets by representing them visually. It allows us to identify patterns, trends, and relationships that may not be apparent when looking at raw numbers or text-based information.
What are some benefits of using data visualization?
Using data visualization provides several benefits, such as improved understanding of information, enhanced decision-making capabilities, effective communication of insights to stakeholders, identification of outliers or anomalies in datasets, and the ability to spot trends or patterns quickly.
What tools can I use for data visualization?
Depending on your requirements and expertise level, numerous tools are available for data visualization. Some popular options include Tableau, Power BI, Python libraries like Matplotlib and Seaborn, R packages like ggplot2, and online platforms such as Google Data Studio.
Are there different types of data visualization?
Yes, various types of data visualization techniques can be used depending on the nature of the data and the insights you want to convey. Examples include bar charts, line graphs, scatter plots, heat maps, treemaps, and network diagrams.
How can I improve my data visualization skills?
To improve your data visualization skills, practice regularly by working with different datasets and experimenting with various visualization techniques. Study examples of compelling visualizations in books or online resources and seek feedback from peers or experts. Stay updated with new tools and techniques emerging in the data visualization domain.