Market basket analysis is a technique used in retail to analyze customer purchase patterns and find connections between products. Businesses can improve their marketing strategies and increase sales by studying what items are frequently bought together.
Predictive market basket analysis, the power of data visualization in data science, and big data technology help companies identify which items are likely to be purchased together, allowing them to optimize product placement and promotional campaigns. This data-driven approach and the boom of people breaking into the data industry will enable businesses to tailor their product groupings and create targeted marketing packages.
This blog post will explore how data mining techniques can boost sales and enhance marketing efforts by analyzing purchase data.
How Does Market Basket Analysis Work?
Market basket analysis is a powerful technique businesses use to uncover hidden patterns and associations in customer purchasing behavior. Market basket analysis helps identify frequently co-purchased items by analyzing transactional data, calculating statistical measures to determine associations, and generating actionable insights for marketing and sales strategies.
Identifying Frequently Co-Purchased Items
One of the primary objectives of market basket analysis is to identify items that are frequently purchased together. This enables businesses to understand customer preferences and create targeted marketing campaigns. By examining transactional data from point-of-sale systems or online purchases, companies can identify which products tend to be bought together in a single transaction. For example:
- A grocery store might discover that customers who buy bread also often purchase milk and eggs.
- An online retailer might find that smartphone customers frequently add phone cases and screen protectors to their cart.
Calculating Statistical Measures to Determine Associations
Once the frequently co-purchased items are identified, market basket analysis calculates statistical measures such as support, confidence, and lift to determine the strength of associations between items. These measures help quantify the likelihood of certain item combinations occurring together.
- Support: Support indicates how frequently an item or item combination appears in transactions. It is calculated by dividing the number of transactions containing the item(s) by the total number.
- Confidence: Confidence measures the reliability of an association rule. It is calculated by dividing the number of transactions containing both items in an association rule by the number of transactions, including the first item.
- Lift: Lift determines how likely two items will be purchased together compared to their probabilities. It is calculated by dividing the confidence value by the support value.
By analyzing these statistical measures, businesses can prioritize associations with high support confidence, lift values, and focus their marketing efforts accordingly.
Generating Actionable Insights for Marketing and Sales Strategies
The ultimate goal of market basket analysis is to generate actionable insights that can drive marketing and sales strategies. This will require data engineering consulting if you’ve not created a data ecosystem. By understanding which products are frequently purchased together, businesses can:
- Cross-Sell and Upsell Opportunities: Identify opportunities to cross-sell or upsell related products based on customer purchasing patterns. For example, a customer who purchases a laptop may also be interested in accessories such as a mouse, keyboard, or laptop bag.
- Bundle Products: Create product bundles by combining commonly purchased items. This encourages customers to buy multiple items simultaneously and increases the average transaction value.
- Targeted Promotions: Tailor promotions and discounts based on customer preferences and associations. Businesses can increase conversion rates and customer satisfaction by offering personalized recommendations or discounts on related items during the checkout process.
Market basket analysis provides valuable insights into consumer behavior, enabling businesses to optimize their product offerings, improve customer experiences, and maximize revenue potential.
Real-Life Examples of Market Basket Analysis
Amazon’s “Customers who bought this also bought” feature
Amazon, the world’s largest online retailer, utilizes market basket analysis to enhance its customers’ shopping experience. One prominent example is their “Customers who bought this also bought” feature. By analyzing the purchasing patterns of millions of customers, Amazon can recommend related products that are frequently purchased together.
This feature serves multiple purposes. Firstly, it helps customers discover complementary items they may not have considered. For instance, if a customer purchases a camera, the recommendations may include accessories such as lenses or memory cards. This not only increases customer satisfaction but also drives additional sales for Amazon.
The “Customers who bought this also bought” feature is a testament to the power of market basket analysis in uncovering hidden relationships between products. It allows Amazon to leverage these insights and provide personalized recommendations to its vast customer base.
Supermarket loyalty programs offering personalized coupons
Supermarkets often employ market basket analysis through their loyalty programs to offer personalized coupons to shoppers. Supermarkets can identify buying patterns and preferences by tracking customers’ purchasing habits and analyzing their transaction data.
These insights enable supermarkets to tailor special offers and discounts based on individual shopping behaviors. For example, if a shopper frequently purchases bread and milk together, the supermarket might send them a coupon for discounted bread when they are buying milk.
By leveraging market basket analysis in loyalty programs, supermarkets can enhance customer loyalty by providing targeted incentives that align with their specific needs and preferences. This not only improves customer satisfaction but also encourages repeat purchases.
Netflix’s movie recommendations based on user viewing history
Netflix revolutionized the entertainment industry by using market basket analysis techniques to offer personalized movie recommendations based on user’s viewing history. By analyzing vast amounts of data from millions of users worldwide, Netflix identifies patterns in viewership behavior and suggests relevant content tailored specifically for each user.
For instance, if a viewer frequently watches action movies, Netflix’s recommendation algorithm will suggest similar genres, such as thrillers or superhero films. This personalized approach enhances the user experience by providing a curated selection of content that aligns with their preferences.
Netflix’s use of market basket analysis in movie recommendations is a prime example of how businesses can leverage customer data to deliver targeted and relevant suggestions. By understanding viewers’ preferences and behavior, Netflix can keep users engaged and satisfied, increasing customer retention.
Market Basket Analysis in Various Industries
Market basket analysis extends beyond e-commerce and entertainment sectors. It has proven valuable in telecommunications, healthcare, and even politics.
In telecommunications, market basket analysis helps identify customer usage patterns. This information enables companies to offer personalized plans or bundles tailored to individual needs. For instance, if a customer frequently uses voice calls and mobile data services, the telecom provider might suggest a package that combines these services at a discounted rate.
In healthcare, market basket analysis aids in identifying associations between medical conditions or treatments. This information assists doctors in making more accurate diagnoses and recommending appropriate treatments based on the patient’s symptoms and medical history.
Even political campaigns utilize market basket analysis techniques to understand voters’ preferences better. By analyzing voter data and identifying correlations between various issues or policies, politicians can tailor their messaging to resonate with specific voter segments effectively.
Other Uses, Terminologies, and Algorithms in Market Basket Analysis
Market basket analysis has proven to be a valuable tool for understanding customer behavior and improving business strategies. In addition to its primary application in retail, there are other uses, terminologies, and algorithms associated with market basket analysis.
Cross-selling and upselling techniques in e-commerce
One of the critical applications of market basket analysis is cross-selling and upselling in e-commerce. Cross-selling involves recommending related products to customers based on their current purchases. For example, if a customer buys a laptop, the retailer may suggest purchasing a laptop bag or accessories. Upselling, on the other hand, involves recommending higher-priced or upgraded versions of products to customers. By analyzing purchase patterns and associations between items, retailers can identify opportunities for cross-selling and upselling.
Pros:
- Increases revenue by encouraging customers to buy additional products.
- Enhances customer satisfaction by providing relevant recommendations.
- Improves customer retention by offering personalized shopping experiences.
Cons:
- Requires accurate data collection and analysis to generate meaningful recommendations.
- This may lead to an overwhelming number of product suggestions if not correctly managed.
- It can potentially annoy customers if recommendations are irrelevant or intrusive.
Lift ratio, conviction, and leverage as additional association rule metrics
In market basket analysis, lift ratio, conviction, and leverage are additional metrics used to evaluate association rules. These metrics provide insights into the strength of relationships between items in a dataset.
- Lift ratio: The lift ratio measures how likely two items will be purchased together compared to their probabilities. A lift ratio greater than 1 indicates a positive correlation between items. For example, suppose the lift ratio between coffee and sugar is 2.5. In that case, it suggests that customers who buy coffee are 2.5 times more likely to purchase sugar than the overall probability of buying sugar.
- Conviction: Conviction quantifies the degree of dependency between items and measures how much one item’s absence affects another’s presence. A conviction value greater than 1 indicates a strong association between items. For instance, if the conviction for purchasing milk without bread is 2.5, it implies that customers who buy milk are 2.5 times more likely not to buy bread than the overall probability of not buying it.
- Leverage: Leverage calculates the difference between the observed frequency of two items occurring together and what would be expected if they were independent. A leverage value greater than 0 signifies a positive association between items. For example, if the leverage for buying apples and oranges is 0.15, it suggests that customers are 0.15 more likely to purchase both fruits together than expected by chance.
Eclat algorithm for vertical market basket analysis
The Eclat (Equivalence Class Transformation) algorithm is an efficient vertical market basket analysis method. Unlike traditional Apriori-based algorithms that focus on finding frequent item sets horizontally across transactions, Eclat works vertically by identifying routine item sets within individual transactions.
Eclat Algorithm Steps:
- Transform transaction data into a vertical format.
- Generate initial sets consisting of single items.
- Calculate support values for each item set based on its occurrence in transactions.
- Prune infrequent itemsets based on minimum support threshold.
- Combine remaining frequent itemsets to form larger combinations.
- Repeat steps 3-5 until no new frequent itemsets can be generated.
Pros:
- Handles large datasets efficiently by focusing on individual transactions.
- Reduces memory requirements compared to horizontal algorithms like Apriori.
- Provides insights into frequently occurring combinations within specific transactions.
Cons:
- Limited scalability when dealing with massive datasets or high-dimensional data.
- May miss infrequent but potentially valuable associations between items.
- Requires careful selection of minimum support threshold to avoid generating too many or too few itemsets.
About Eclat; From Wiki
Eclat[11] (alt. ECLAT, which stands for Equivalence Class Transformation) is a backtracking algorithm that traverses the frequent itemset lattice graph in a depth-first search (DFS) fashion. Whereas the breadth-first search (BFS) traversal used in the Apriori algorithm will end up checking every subset of an itemset before reviewing it, DFS traversal corresponds to larger itemsets. It can save on checking the support of some of its subsets by the downward-closer property. Furthermore, it will almost certainly use less memory as DFS has a lower space complexity than BFS.
Step-by-step Guide for Performing Market Basket Analysis in Python
Installing Necessary Libraries like Pandas and MLxtend
We must establish a few essential libraries for the model to perform market basket analysis in Python. One of the tools for data manipulation and analysis is Pandas, a popular model. Another vital library for machine learning algorithms is MLxtend, which offers various models, including the Apriori algorithm we will use for market basket analysis.
Here are the steps to install these libraries:
- Open your command prompt or terminal.
- Type pip install pandas model and press Enter to install the Pandas library.
- Once Pandas is installed, type
pip install mlxtend
and press Enter to install the MLxtend library.
Loading Transaction Data into a DataFrame
After installing the necessary libraries, we can load our transaction data into a DataFrame using the model. A DataFrame is a two-dimensional tabular data structure provided by the Pandas library. It serves as a model for organizing and analyzing data efficiently.
Here’s how you can load transaction data into a DataFrame using a model.
- To import the required libraries for your Python script, add the following lines of code at the beginning of your script: model. Import.
import pandas as pd
from mlxtend.preprocessing import TransactionEncoder
- Read your transaction data from a file or any other source using Pandas’ read_csv() function. This function is an essential tool for analyzing and manipulating data in the model.
df = pd.read_csv('transaction_data.csv')
- Ensure that your transaction data is adequately structured, with each row representing a unique transaction and each column representing an item purchased during that transaction. This structured format is essential for accurately modeling and analyzing transaction data.
- Convert your transaction data into a list of lists format expected by MLxtend’s Apriori algorithm:
transactions = df.values.tolist()
Applying the Apriori Algorithm to Find Frequent Itemsets
Now that we have loaded our transaction data into a DataFrame, we can apply the Apriori algorithm from the MLxtend library to find frequent item sets. Frequent itemsets are sets of items that occur together in many transactions.
Here’s how you can apply the Apriori algorithm:
- Create an instance of the
TransactionEncoder
class from MLxtend:
te = TransactionEncoder()
- Use the
fit()
method to encode your transaction data into a one-hot encoded format:
te_ary = te.fit(transactions).transform(transactions)
- Convert the one-hot encoded data back into a DataFrame using Pandas:
df_encoded = pd.DataFrame(te_ary, columns=te.columns_)
- Apply the Apriori algorithm to find frequent itemsets with a specified minimum support threshold:
from mlxtend.frequent_patterns import apriori
frequent_itemsets = apriori(df_encoded, min_support=0.05, use_colnames=True)
- Optionally, you can filter the frequent itemsets based on other criteria, such as minimum or maximum length, using Pandas’ dataframe operations.
By following these steps, you can perform market basket analysis in Python using the Apriori algorithm and extract valuable insights about which items customers frequently purchase together.
Importance of Market Basket Analysis in SEO Content Writing
DEV3LOP started and continues to be an SEO-focused content-writing business. We create free content for informative researchers, and that helps us heighten our technical services. In the day of AI, creating content is becoming more accessible and comprehensive, and we spend a lot of time using AI, ML, or introductory statistics.
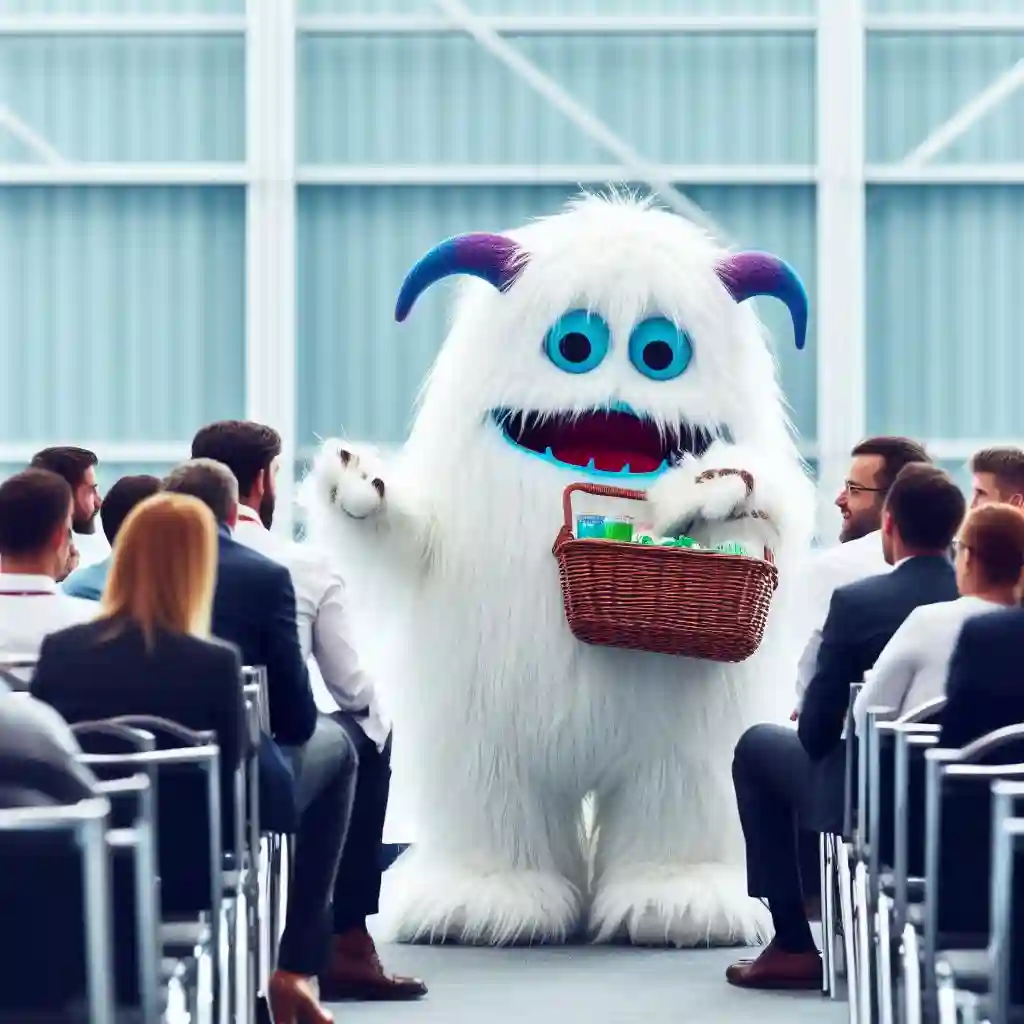
Market basket analysis is a proper data mining technique for SEO content writing. It helps identify trends and decide which products to promote. Studies show that it can increase sales by up to 15%. Improving user experience and search engine rankings plays a crucial role in digital success. It involves data mining, feature extraction, and clustering to enhance product recommendations and cross-selling opportunities. It can be used in different industries, like the camera industry.
Optimizing Content for Better User Experience
Market basket analysis helps SEO content writers understand customer purchasing behavior by analyzing data and identifying patterns. This information can be used to create more relevant and engaging content that meets the target audience’s needs, improving the user experience.
- Pro: Increased user engagement and satisfaction.
- Pro: Higher conversion rates as users find relevant information.
- Example: A blog post about “10 Essential Tools for Home Gardening” could be optimized by including product recommendations such as gardening gloves, pruners, or fertilizer. Similarly, a blog post about “The Top 5 Cameras for Photography Enthusiasts” could be optimized by including recommendations for popular camera models with advanced attributes and features. Additionally, data mining techniques and advanced analytics can be applied to analyze consumer preferences and conduct predictive market basket analysis to identify which camera accessories are commonly purchased together.
Enhancing Product Recommendations and Cross-Selling Opportunities
One of the critical benefits of market basket analysis is its ability to uncover patterns in customer buying behavior through data mining. This technique allows for identifying clusters based on customer attributes, providing valuable insights into consumer preferences and trends. This information can enhance product recommendations and cross-selling opportunities within SEO content by utilizing the attributes, percent, clusters, and models. By understanding which products are frequently purchased together, writers can strategically promote related items to increase sales and customer satisfaction. This can be achieved using a classification model to identify clusters of products often bought together, allowing writers to target these specific groups and increase sales by x percent.
- Pro: Increased revenue through cross-selling opportunities.
- Pro: Improved customer experience by suggesting complementary products.
- Example: An article on “The Best Skincare Routine” could include links or suggestions for related skincare products like moisturizers, serums, or cleansers. These products can be classified into different categories based on their ingredients and benefits. Using a classification model, skincare enthusiasts can quickly identify the best products for their skin concerns. Additionally, some skincare routines may combine products from different clusters, such as exfoliators or masks, to achieve optimal results. Just like how other fish species belong to various clusters based on their characteristics, skincare products can also be grouped based on
Improving Keyword Targeting and Search Engine Rankings
Market basket analysis provides valuable insights into keyword targeting by identifying commonly associated terms used in customer searches. This classification model can help businesses understand the patterns and relationships between different search terms by analyzing clusters of related keywords. For example, if a customer searches for “fish,” the model can identify other frequently searched terms such as “aquarium,” “seafood,” and “fishing.” This information can be used to optimize keyword targeting and improve search engine optimization strategies. By incorporating fish, classification, and model keywords into SEO content, writers can improve search engine rankings and attract more organic website traffic. Understanding the relationships between different products allows for creating targeted content that aligns with user search intent. This understanding is crucial for developing a practical model that caters to user needs and preferences. By identifying and analyzing these relationships, businesses can optimize their content strategy to serve their target audience better.
- Pro: Higher visibility in search engine results pages.
- Pro: Increased organic traffic and brand exposure.
- Example: A blog post about “Healthy Breakfast Ideas” could incorporate keywords related to frequently used ingredients together, such as “oats and berries” or “avocado and toast.”
Exploring the FP-Growth Algorithm in Market Basket Analysis
The FP-Growth algorithm is a powerful tool used in market basket analysis to efficiently mine frequent itemsets from large datasets. This algorithm utilizes a tree-based structure known as the FP-tree, allowing faster processing and handling of sparse transaction data.
Efficiently mining frequent itemsets from large datasets
One of the critical challenges in market basket analysis is dealing with large datasets that contain a vast number of transactions. The traditional approach of using an Apriori algorithm can be time-consuming and computationally expensive. However, the FP-Growth algorithm offers a more efficient solution.
The FP-Growth algorithm creates an FP tree, which represents the frequent patterns found in the dataset. This tree structure allows for faster identification of frequent itemsets without generating candidate itemsets explicitly. By eliminating the need for candidate generation, the FP-Growth algorithm significantly reduces computational overhead.
Utilizing a tree-based structure for faster processing
The main advantage of using the FP-tree structure is its ability to speed up the mining process. The construction of an FP-tree involves two passes over the dataset: one pass to determine frequent items and build a header table and another pass to construct the actual tree.
Once constructed, mining frequent item sets becomes much faster because it only requires traversing paths in the tree corresponding to specific items or sets of items. This eliminates the need to generate all possible combinations, improving efficiency.
Handling sparse transaction data effectively
Sparse transaction data refers to datasets where most transactions contain only a small subset of available items. Traditional algorithms struggle with this type of data because they generate many candidate item sets that are unlikely to be frequent.
The FP-Growth algorithm excels at handling sparse transaction data due to its compact representation using an FP tree. Since infrequent or non-existent items are pruned during construction, only relevant information is retained in memory. This reduces the memory footprint and improves overall performance.
Pros of using the FP-Growth algorithm in market basket analysis:
- Efficiently mines frequent itemsets from large datasets, reducing computational overhead.
- Utilizes a tree-based structure for faster processing, improving efficiency.
- Handles sparse transaction data effectively by pruning irrelevant information.
Cons of using the FP-Growth algorithm in market basket analysis:
- Requires additional preprocessing steps to transform the dataset into a suitable format for constructing an FP-tree.
- It may not be as effective when dealing with tiny datasets or highly skewed item distributions.
Creating Association Rules for Market Basket Analysis
In market basket analysis, the goal is to establish relationships between items in a transactional dataset. This is achieved through association rules, which provide insights into item combinations that frequently co-occur. By analyzing these associations, businesses can gain valuable insights to optimize their product placement, cross-selling strategies, and promotional campaigns.
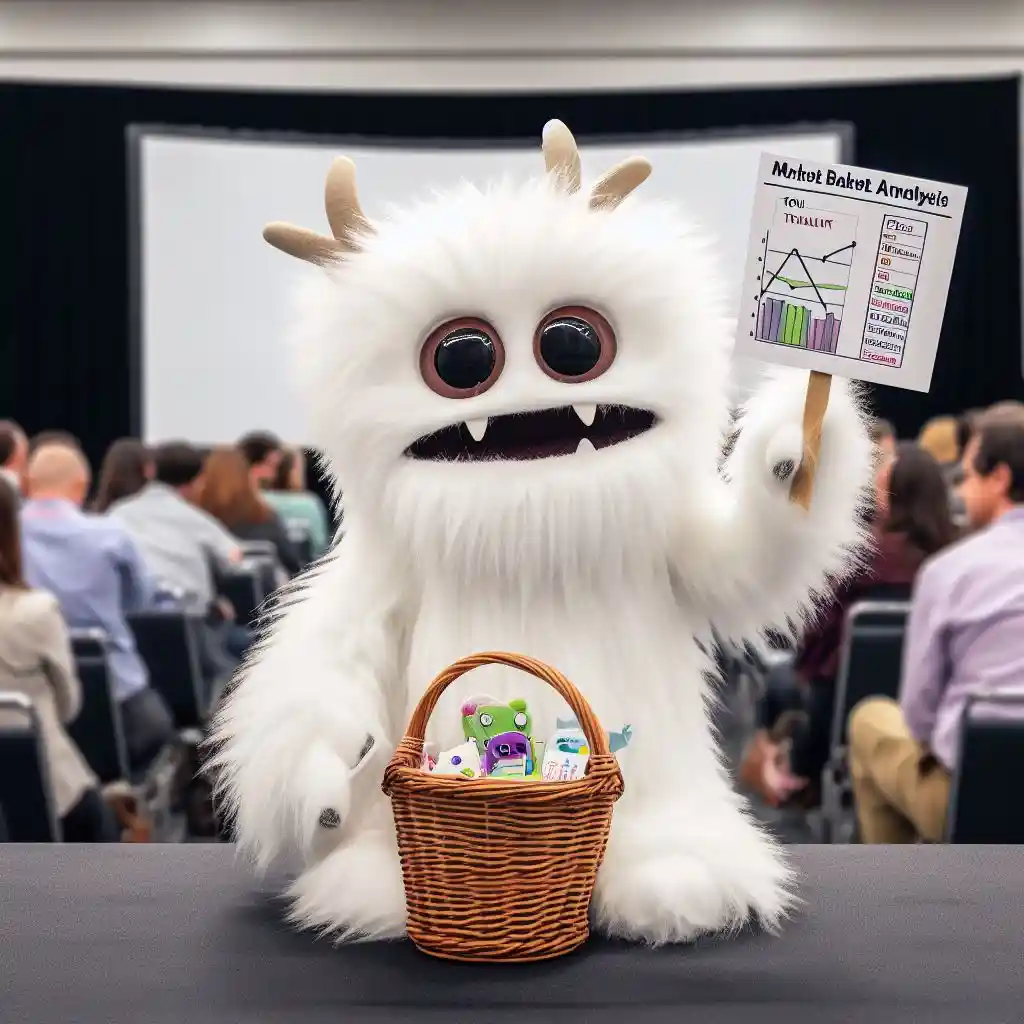
Establishing Relationships Using Support, Confidence, and Lift Metrics
To create association rules, we utilize metrics such as support, confidence, and lift.
- Support measures the frequency of an item set or rule in a dataset. It indicates how often a particular combination of items occurs together in transactions.
- Confidence determines the reliability of a rule by measuring the conditional probability that item B is purchased, given that item A has already been purchased. It helps identify how likely it is for one item to be bought when another item is already present in the market basket.
- Lift quantifies the strength of an association rule by comparing its actual occurrence with what would be expected if there was no relationship between the items. Lift values greater than 1 indicate positive associations, while values less than 1 indicate negative associations.
By calculating these metrics using algorithms like Apriori or FP-Growth, we can identify meaningful associations within a dataset.
Setting Thresholds to Filter Out Insignificant Rules
When generating association rules, it’s essential to set thresholds for support, confidence, and lift to filter out insignificant rules. These thresholds help ensure that only meaningful and actionable rules are considered.
Setting too low thresholds may result in numerous trivial or uninteresting rules that do not provide much value. On the other hand, setting thresholds too high may eliminate potentially applicable rules from consideration.
It’s essential to strike a balance based on domain knowledge and business requirements when determining threshold values. Experimentation with different threshold levels can help identify suitable settings for each metric.
Interpreting Association Rule Results for Actionable Insights
Once the association rules have been generated, it’s crucial to interpret the results to derive actionable insights. Here are some key considerations:
- Support and Confidence: Focus on rules with high support and confidence values. These rules indicate strong associations and can guide decision-making processes.
- Lift: Look for rules with lift values significantly above 1. These rules represent meaningful relationships between items more likely to be purchased together than expected by chance alone.
- Rule Length: Consider the length of the association rule. More extended rules may provide more specific insights into item combinations, while shorter rules may offer broader patterns.
- Domain Knowledge: Combine the statistical analysis of association rules with domain knowledge to uncover hidden patterns and make informed business decisions.
By analyzing and interpreting association rule results, businesses can gain valuable insights into customer behavior, optimize product offerings, improve cross-selling strategies, and enhance overall sales performance.
Critical Insights from Market Basket Analysis
Popular Product Combinations or Bundles
Market basket analysis provides valuable insights into popular product combinations or bundles that customers tend to purchase together. By analyzing transaction data, retailers can identify which items are frequently bought together in a single shopping trip. This information allows businesses to leverage these associations and create effective marketing strategies.
For example:
- A grocery store may find that customers who purchase bread are highly likely to buy milk and eggs. With this knowledge, the store can strategically place these items nearby to encourage additional purchases.
- Online retailers often display recommended products based on market basket analysis. For instance, if a customer adds a camera to their cart, the retailer might suggest complementary accessories such as lenses or memory cards.
By understanding popular product combinations, businesses can optimize their product groupings and promotions to increase sales and enhance the overall customer experience.
Seasonal Purchasing Patterns or Trends
Market basket analysis can uncover seasonal purchasing patterns or trends within the retail industry. By examining transaction data over different periods, businesses can identify shifts in consumer behavior and tailor their strategies accordingly.
For instance:
- During the holiday season, customers may be more inclined to purchase gift sets or themed bundles. Retailers can capitalize on this trend by creating special holiday promotions targeted at specific customer segments.
- In warmer months, there may be an increase in sales of outdoor equipment and picnic essentials. By recognizing this seasonal pattern, retailers can adjust their inventory levels and marketing campaigns accordingly.
Understanding seasonal purchasing patterns enables businesses to align their offerings with customer preferences at different times of the year, maximizing sales opportunities and enhancing customer satisfaction.
Cross-Category Associations for Targeted Promotions
Market basket analysis not only reveals associations within a single category but also identifies cross-category associations. This means that customers frequently purchase certain products from different categories.
For example:
- A study might show that customers who buy diapers will also likely purchase baby wipes and formula. By leveraging this cross-category association, retailers can create targeted promotions that offer discounts or incentives on related products to encourage additional purchases.
- Similarly, a customer who buys running shoes may also be interested in athletic apparel or fitness accessories. By understanding these cross-category associations, retailers can tailor their marketing campaigns to promote relevant products and increase the average basket size.
By utilizing cross-category associations, businesses can optimize their promotional strategies by offering customers personalized recommendations and enticing them to explore complementary products.
Understanding Market Basket Analysis from the Customers’ Perspective
Market basket analysis provides valuable insights into customer purchasing patterns and behavior. By analyzing customers’ purchase histories, retailers can gain a deeper understanding of their preferences and needs.
Discovering Complementary Products that Enhance User Experience
One of the critical advantages of market basket analysis is its ability to uncover complementary products that enhance the user experience. By examining the items frequently purchased together, retailers can identify product combinations that complement each other. For example:
- Customers who purchase a laptop may also need a laptop bag or accessories, such as a mouse or keyboard.
- Someone buying a camera might be interested in lenses, memory cards, or camera cases.
By identifying these associations, retailers can offer bundled deals or recommend related products to enhance the overall shopping experience for customers. This not only increases customer satisfaction but also encourages them to make additional purchases.
Providing Personalized Recommendations Based on Past Purchases
Market basket analysis allows retailers to provide personalized recommendations based on customers’ past purchases. By leveraging data on previous transactions, retailers can understand individual preferences and tailor product suggestions accordingly. This level of personalization enhances the shopping experience by offering relevant and targeted recommendations.
For instance:
- A customer who frequently buys organic food products might receive recommendations for new organic brands or similar healthy alternatives.
- An individual who regularly purchases skincare items could be suggested new skincare products based on their specific skin type or concerns.
These personalized recommendations create value for customers as they feel understood and catered to by the retailer. It also saves time for customers by presenting them with options that align with their interests and preferences.
Influencing Buying Decisions through Suggestive Selling Techniques
Market basket analysis empowers retailers to influence buying decisions through suggestive selling techniques. By analyzing customer purchasing patterns, retailers can identify opportunities to upsell or cross-sell products. For example:
- A customer purchasing a smartphone may be offered an extended warranty or additional accessories.
- Someone buying a dress might receive recommendations for matching shoes or accessories.
By strategically suggesting complementary or upgraded products during the purchase process, retailers can increase the average transaction value and maximize revenue. This technique also benefits customers by providing options that enhance their original purchase and meet their needs more comprehensively.
Data Preparation and Preprocessing for Market Basket Analysis
To perform market basket analysis effectively, it is crucial to prepare and preprocess the data appropriately. This ensures the data is in a suitable format for mining association rules and extracting meaningful insights. Let’s explore the critical steps in data preparation and preprocessing for market basket analysis.
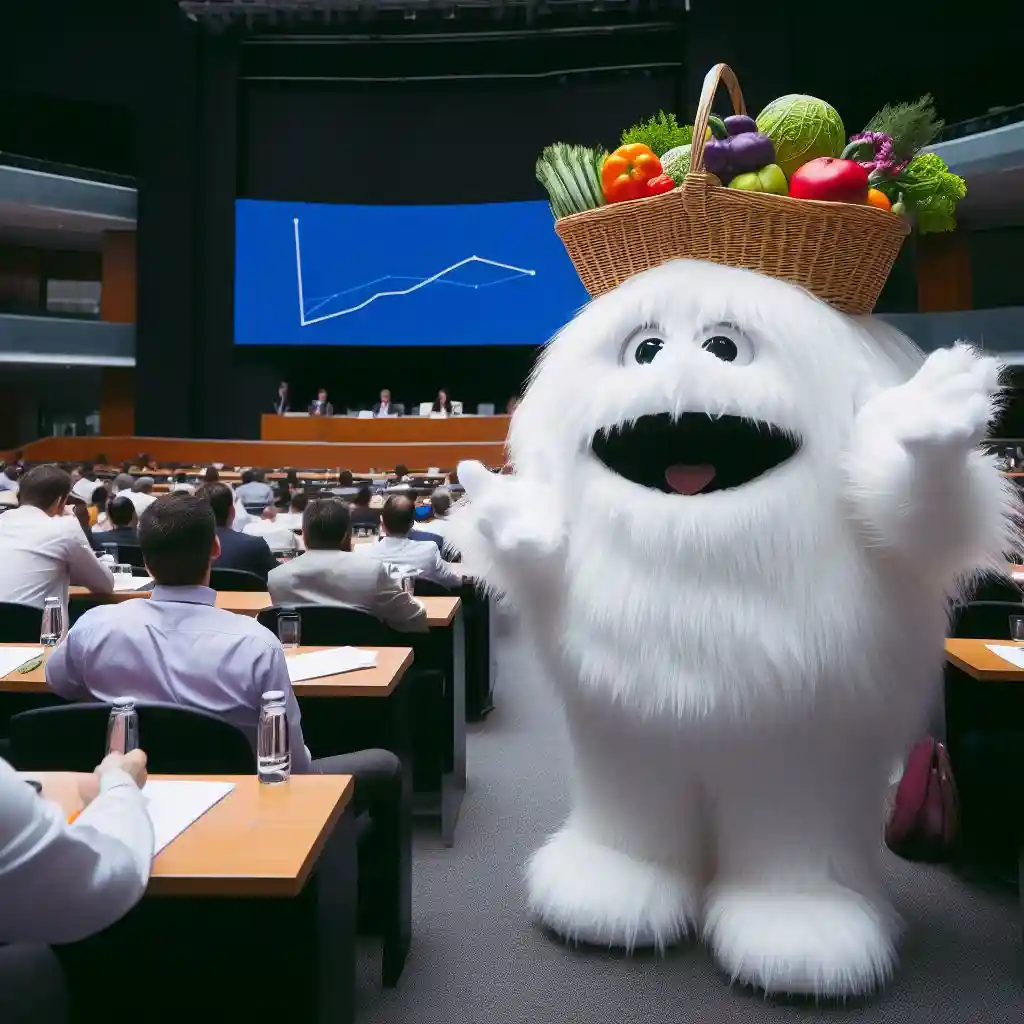
Removing Duplicate Transactions or Outliers
A critical step in data preparation is removing duplicate transactions or outliers from the dataset. The same transactions can skew the results of market basket analysis by artificially inflating the support and confidence values of itemsets. Similarly, outliers can introduce noise and distort the patterns present in the data.
To address this issue, data scientists need to carefully examine the dataset and identify any duplicate transactions or outliers. These can be removed using various statistical methods or domain knowledge-based approaches. By eliminating duplicates or outliers, we ensure that our analysis is based on clean and reliable data.
Transforming Data into a Suitable Format
Another critical aspect of data preparation for market basket analysis is transforming the raw purchase data into a suitable format. This typically involves converting the transactional data into a binary format where each row represents a unique transaction, and each column represents an item purchased.
This transformation allows us to apply various data mining techniques, including association rule mining algorithms, to uncover interesting patterns within the dataset. By representing transactions as binary vectors, we can efficiently identify frequent item sets and generate association rules that reveal relationships between items.
Handling Missing Values Appropriately
Dealing with missing values is another important consideration when preparing data for market basket analysis. Missing values can arise for various reasons, such as incomplete records or errors during data collection. Ignoring missing values or imputing them without consideration can lead to biased results.
To handle missing values appropriately, several strategies can be employed depending on the nature of the problem at hand. Some common approaches include removing transactions with missing values, imputing missing values based on statistical measures such as mean or median, or using advanced techniques like multiple imputation.
By addressing missing values effectively, we ensure that our analysis is based on complete and reliable data, leading to more accurate insights and actionable recommendations.
Types of Market Basket Analysis Techniques
Market Basket Analysis is a powerful technique used in data mining to uncover associations and patterns between items purchased together. Several techniques are available for conducting Market Basket Analysis, each with strengths and limitations. Let’s explore three popular techniques: Traditional association rule mining (Apriori algorithm), Frequent pattern growth (FP-Growth algorithm), and Sequential pattern mining (PrefixSpan algorithm).
Traditional Association Rule Mining (Apriori Algorithm)
The Apriori algorithm is one of the most widely used techniques for Market Basket Analysis. It follows a two-step process:
- Generating frequent itemsets: The algorithm scans the transaction database to identify frequently occurring itemsets that meet a user-defined minimum support threshold. These frequent itemsets represent combinations of items that appear together frequently enough to be considered significant.
- Generating association rules: Once the frequent itemsets are identified, the Apriori algorithm generates association rules by examining the subsets of these itemsets. An association rule consists of an antecedent (the items on the left-hand side) and a consequent (the items on the right-hand side). The algorithm calculates various metrics, such as support, confidence, and lift, to measure the strength of these rules.
Pros of using the Apriori Algorithm:
- Widely adopted and well-established technique in Market Basket Analysis.
- Can handle large datasets efficiently.
- Provides interpretable results in terms of association rules.
Cons of using the Apriori Algorithm:
- Computationally expensive when dealing with large numbers of candidate itemsets.
- Requires multiple passes over the dataset, which can be time-consuming.
- Prone to generating a high number of spurious or irrelevant rules.
Frequent Pattern Growth (FP-Growth Algorithm)
The FP-Growth algorithm is an alternative approach to traditional association rule mining that addresses some limitations associated with Apriori. Instead of generating candidate itemsets, FP-Growth constructs a compact data structure called an FP-Tree to represent the transaction database.
- Building the FP-Tree: The algorithm scans the transaction database once to construct the FP-Tree. This tree structure allows for an efficient and compact representation of frequent itemsets in the dataset.
- Mining frequent patterns: Once the FP tree is built, regular patterns can be extracted by recursively traversing the tree. This process eliminates the need for generating candidate itemsets, resulting in faster performance than Apriori.
Pros of using the FP-Growth Algorithm:
- Efficient and scalable technique for large datasets.
- Eliminates the need for generating candidate itemsets, reducing computation time.
- Can handle both dense and sparse datasets effectively.
Cons of using the FP-Growth Algorithm:
- Requires additional memory to store the FP-Tree structure.
- It may not perform as well as Apriori when dealing with high-dimensional datasets.
- Limited interpretability compared to traditional association rule mining.
Sequential Pattern Mining (PrefixSpan Algorithm)
Sequential pattern mining is a variant of Market Basket Analysis that focuses on capturing sequential associations between items. It is beneficial when analyzing transactional data with a temporal component, such as customer purchase histories or web clickstreams.
- Identifying frequent sequential patterns: The PrefixSpan algorithm scans sequences of transactions to identify frequently occurring subsequences that meet a user-defined minimum support threshold. These subsequences represent sequential patterns that occur together frequently enough to be considered significant.
- Generating association rules: Once frequent sequential patterns are identified, association rules can be caused by examining subsets of these patterns similar to traditional association rule mining techniques.
Pros of using Sequential Pattern Mining:
- Captures temporal dependencies and order in which items are purchased or accessed.
- It helps analyze customer behavior over time or identify browsing patterns on websites.
- Can uncover hidden insights not easily discovered through other techniques.
Cons of using Sequential Pattern Mining:
- Requires sequential data with a temporal component.
- Computationally expensive for large datasets.
- Limited interpretability compared to traditional association rule mining.
Conclusion: Key Insights from Market Basket Analysis
In conclusion, market basket analysis is a powerful technique that provides valuable insights into customer behavior and purchasing patterns. By analyzing the items that customers frequently purchase together, businesses can uncover hidden relationships and make informed decisions to optimize their marketing strategies. Through this analysis, SEO content writers can identify popular product combinations and create compelling content that promotes cross-selling and upselling opportunities.
To perform market basket analysis effectively, it is crucial to follow a step-by-step guide using Python and explore algorithms like FP-Growth. This allows for creating association rules that reveal essential connections between products. Moreover, understanding market basket analysis from the customers’ perspective enables businesses to tailor their offerings and enhance the shopping experience.
By implementing data preparation and preprocessing techniques, businesses can ensure accurate results in their market basket analysis. Being familiar with different types of market basket analysis techniques helps in selecting the most appropriate approach for specific business goals. Real-life examples illustrate how this method has been successfully applied across various industries.
Incorporating market basket analysis into your business strategy empowers you to make data-driven decisions that improve customer satisfaction, increase revenue, and drive long-term success. Start leveraging this powerful tool today!
FAQs
How does market basket analysis benefit e-commerce companies?
Market basket analysis benefits e-commerce companies by providing insights into customer purchasing patterns. It helps identify products frequently bought together, allowing businesses to optimize their cross-selling and upselling strategies. This can lead to increased sales revenue and improved customer satisfaction.
What is the significance of association rules in market basket analysis?
Association rules play a crucial role in market basket analysis as they reveal relationships between items purchased by customers. Businesses can use these rules to understand which products are commonly associated with each other and make informed decisions about product placement, promotions, or bundling strategies.
Can small businesses benefit from market basket analysis?
Yes, small businesses can significantly benefit from market basket analysis. It allows them to gain insights into their customers’ preferences and purchasing behavior, enabling them to optimize their product offerings and marketing strategies. By understanding which products are frequently bought together, small businesses can enhance the customer experience and increase sales.
Are there any limitations or challenges in conducting market basket analysis?
While market basket analysis is a powerful technique, it does have some limitations. One challenge is dealing with large datasets that contain numerous transactions and items, which can impact computational efficiency. Interpreting the results of market basket analysis requires domain knowledge and expertise to make meaningful business decisions.
Can market basket analysis be applied to non-retail industries?
Yes, market basket analysis can be applied to non-retail industries as well. For example, it can be used in healthcare to identify patterns in patient treatments or medication prescriptions. In telecommunications, it can help understand calling patterns or service bundling opportunities. The principles of market basket analysis can be adapted to various industries where transactional data exists.