Data mining allows people to fix, find, replace, update, and report on their findings. So, imagine stumbling upon an old attic filled with forgotten treasures after looking through the entire house.
Finding answers to your questions is like mining these treasures, and in this era of AI, getting new information can be exciting or inspire unique ideas. Yes, AI is making our lives easier. However, we must all gain the fundamentals, which may be all that’s necessary to implement before we start progressing to AI data mining technologies.
Data miners uncover valuable treasures and help you access those treasures in meaningful ways. This is similar to the world of database queries and building dashboards.
Data mining involves mining meaningful insights from raw data in the cloud or on-premise, like finding gems in your office space. Data mining is extracting and discovering patterns in large data sets involving methods at the intersection of machine learning, statistics, and database systems (wiki definition).
This blog post will explore how to start data mining and the essential steps to begin your journey. Data mining is crucial for leveraging company data effectively. By following the suitable model, you can unlock valuable insights and make informed decisions based on the information you gather through data mining. Our company provides insights on using cloud technology for efficient data mining and has a decade of experience using on-premise servers.
So, if you’re ready to use the cloud to unlock the hidden potential within your company’s data and transform it into valuable knowledge and actionable insights, let’s dive right in! Start mining the power of the cloud today.
About The White Monster, Who is a Data Mining Guru
Once upon a time, there was a furry white monster named Fluffy in a land full of secrets and hidden treasures. Fluffy loved to explore and find valuable things.
Fluffy searched for hidden riches everywhere, from the sunny fields to the dark caves. He faced challenges like puzzles and rugged paths but never gave up.
One day, after weeks of searching, Fluffy found a unique treasure chest. Inside were jewels and gold, the joy of the creatures in the forest, the rivers’ songs, and the old trees’ wisdom. It was like a collection of beautiful experiences.
Fluffy shared his treasure and stories with his friends and the world. He realized that the best part of his adventure was not the treasure but the journey, the fun of exploring, and the friends he made along the way.
Introduction to “Data Mining How To”
If you’re new to data mining or looking to enhance your skills using the company’s model, this guide is for you. Data mining, also known as mining, refers to the process of extracting valuable insights and patterns from large datasets. This is a crucial model for any company to use to gain a competitive advantage. The mining process involves analyzing vast amounts of data using various techniques to uncover hidden relationships, trends, and patterns that can be used to make informed decisions. This mining process utilizes a model to extract valuable insights efficiently.
Benefits of Learning Data Mining Techniques
Learning about data mining techniques, such as mining, can offer numerous benefits beyond just acquiring knowledge from forgotten data, public data, and internal data.
Key advantages
- Unlocking Valuable Insights: By understanding the mining process and how to harvest data, you can gain valuable insights into your business operations, finances, accounts, human resources, or research projects. This understanding allows you to develop a model for extracting the most relevant information. The data mining process, carried out by data mining specialists, can utilize large data sets to uncover valuable insights. These insights, derived from current data, can aid in identifying growth opportunities, detecting potential risks, and making better-informed decisions.
- Identifying Patterns and Trends: Data mining allows you to discover hidden patterns and trends within your datasets that might not be apparent at first glance. This current data can be crucial in data mining, as it helps identify customer preferences, market trends, or anomalies that require attention. Whether analyzing large data sets or attending a data science boot camp, access to current data is essential.
- Improving Decision-Making: With the ability to analyze data efficiently, you can make more accurate predictions and informed decisions based on evidence rather than intuition alone. Using large data sets in the data mining process can improve operational efficiency, cost savings, and overall outcomes at a data science boot camp.
- Enhancing Problem-Solving Abilities: Data mining equips you with powerful tools for problem-solving by enabling you to identify underlying causes or factors contributing to specific issues or challenges. You can develop effective strategies and solutions by understanding these factors in the context of data science and data sets.
Unlocking Valuable Insights with Data Mining How To
“Data Mining How To” is a comprehensive guide that empowers individuals like yourself with the knowledge and skills to unlock valuable insights from your data. Whether working on a personal data science project, conducting research using data sets, or seeking ways to optimize your business operations, this guide will provide practical tips and techniques to achieve your goals.
What to Expect from This Comprehensive Guide
In this guide, we will cover various aspects of data mining. You can expect to learn about:
- Preparation: We will discuss the essential steps involved in preparing for a data mining project. This includes identifying the problem or question you want to address, gathering relevant data, and ensuring its quality and integrity.
- Methods and Techniques: We will explore different data mining methods and techniques that can be applied to extract meaningful insights from your datasets. This may include classification, clustering, association rule mining, and more.
- Implementation: You’ll gain insights into how to implement these techniques using popular tools and programming languages such as Python or R. We’ll provide step-by-step instructions and code examples to help you get started.
- Pattern Recognition: Understanding patterns is at the core of data mining. We’ll delve into various pattern recognition algorithms and approaches that can assist you in uncovering hidden patterns within your datasets.
By following this comprehensive guide on “Data Mining How To,” you’ll be equipped with the knowledge and skills to tackle real-world problems effectively using data mining techniques.
Understanding the concept and applications of data mining
Define what data mining is and its significance in various industries
Data mining is a powerful technique to extract valuable insights and patterns from large datasets. It involves analyzing vast amounts of data to discover hidden relationships, trends, and patterns that can be used for decision-making and problem-solving. In today’s data-driven world, where organizations collect massive amounts of information, data mining is crucial in uncovering valuable knowledge.
The significance of data mining spans across various industries. For instance, in retail, it helps identify customer buying patterns, allowing businesses to personalize marketing campaigns and optimize inventory management. In healthcare, data mining aids in detecting disease outbreaks, predicting patient outcomes, and improving treatment plans. Financial institutions use data mining to detect fraudulent activities by analyzing transactional data and identifying suspicious patterns. Data mining has become indispensable for gaining actionable insights from telecommunications to manufacturing to social media platforms.
Explore real-world examples of how data mining is used for decision-making and problem-solving
Data mining has numerous practical applications that have revolutionized decision-making processes across industries. Let’s delve into some real-world examples:
- Customer Segmentation: Retailers leverage data mining techniques to segment their customer base based on demographics, purchasing behavior, or preferences. This allows them to tailor marketing strategies for each segment more effectively.
- Churn Prediction: Telecommunication companies analyze customer usage patterns using data mining algorithms to predict which customers are likely to churn (cancel their subscriptions). By identifying these customers early on, they can implement retention strategies.
- Fraud Detection: Financial institutions employ sophisticated algorithms to detect fraudulent transactions by analyzing historical transactional records for unusual patterns or anomalies.
- Recommendation Systems: Online streaming platforms like Netflix use collaborative filtering techniques through data mining algorithms to recommend personalized content based on user’s viewing history and preferences.
- Medical Research: Data mining analyzes large datasets and identifies potential disease risk factors or uncover patterns that can lead to new treatment discoveries.
Understand how patterns, relationships, and trends are discovered through data mining algorithms.
Data mining algorithms are critical in discovering patterns, relationships, and trends within datasets. These algorithms use statistical techniques to analyze the data and extract meaningful insights. Here’s a glimpse into how these discoveries are made:
- Association Rule Mining: This algorithm discovers relationships between items in a dataset. For example, it might reveal that customers who purchase diapers are also likely to buy baby wipes.
- Clustering: Clustering algorithms group similar data points based on their attributes or characteristics. For instance, customer segmentation can be achieved by clustering customers with similar purchasing behavior.
- Classification: Classification algorithms assign predefined classes or labels to data instances based on their attributes. For example, an algorithm could classify emails as spam or non-spam based on specific keywords or patterns.
- Regression Analysis: Regression analysis helps predict numerical values based on historical data patterns and relationships between variables. It enables forecasting future outcomes based on existing trends.
Learn about predictive modeling and its role in extracting valuable information from large datasets.s
Predictive modeling is a critical component of data mining that involves creating mathematical models to predict future outcomes or behaviors based on historical data patterns. It utilizes various techniques such as regression analysis, decision trees, neural networks, and support vector machines.
The process of predictive modeling typically involves the following steps:
- Data Collection: Gathering relevant data from various sources to build a comprehensive dataset for analysis.
- Data Preprocessing: Cleaning and transforming the raw data by removing outliers, handling missing values, normalizing variables, etc.
Techniques involved in Data Mining
Several techniques can be employed to uncover hidden patterns and insights within datasets. Explore popular data mining techniques and understand how they contribute to practical analysis.
Also, explore another great monster image.
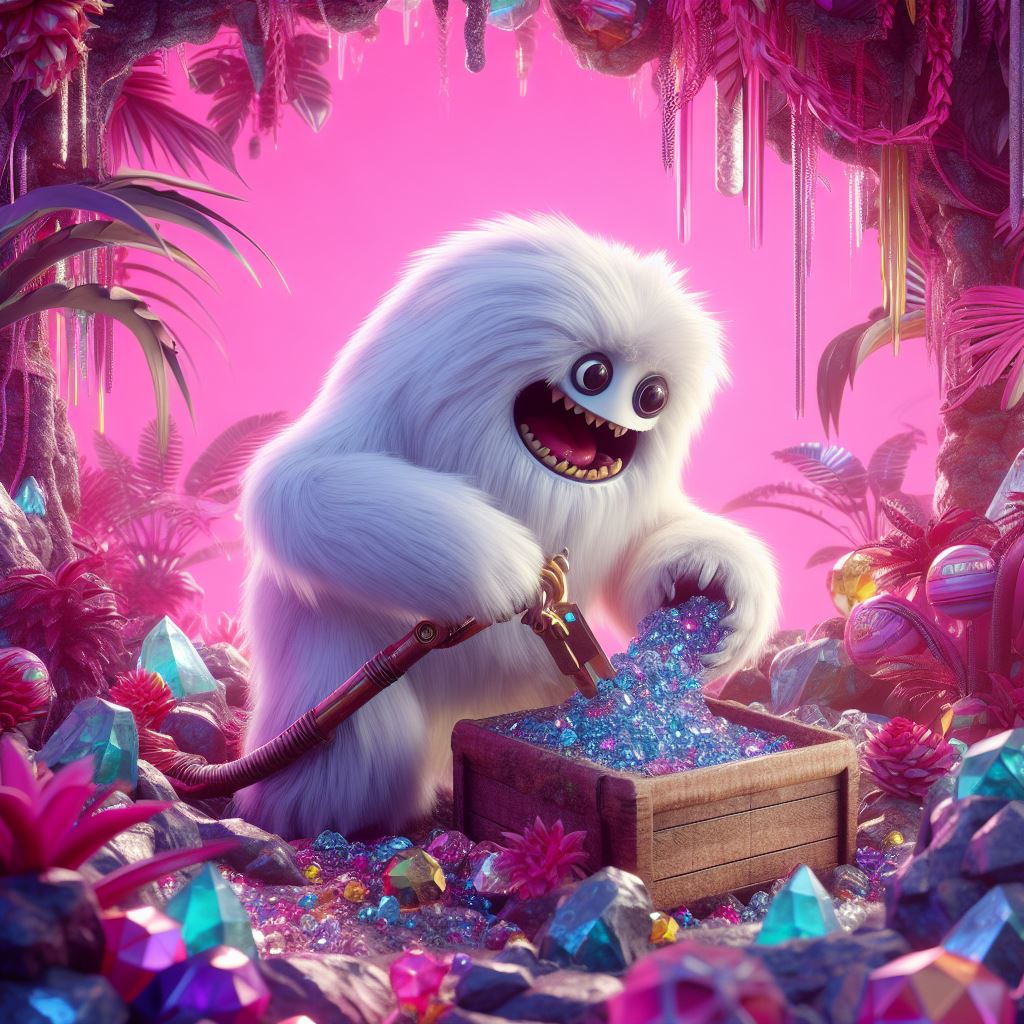
Familiarize yourself with popular techniques used in data mining
Data mining involves classification, clustering, association rules, and anomaly detection. Each technique plays a crucial role in extracting valuable information from raw data.
- Classification: This technique categorizes data into predefined classes or groups based on specific attributes. It helps predict future outcomes or classify new instances based on existing patterns.
- Clustering: Clustering groups similar objects based on their characteristics or similarities. It helps identify natural clusters within the data and discover relationships that may not be apparent initially.
- Association Rules: This technique focuses on finding associations or relationships between different items in a dataset. It is commonly used for market basket analysis, where co-occurrence patterns among products are identified.
- Anomaly Detection: Anomaly detection aims to identify unusual or abnormal observations that deviate significantly from the expected behavior. It is helpful for fraud detection, network intrusion detection, and other applications where identifying outliers is critical.
Understand how each technique contributes to uncovering hidden patterns within datasets.
Each data mining technique has its strengths and applications. By understanding these techniques, you can leverage their capabilities to extract meaningful insights from your data.
- Classification enables you to build predictive models to classify new instances into predefined classes. For example, it can help predict whether a customer will churn based on historical customer behavior.
- Clustering allows you to discover inherent structures or groups within your dataset without knowledge of the classes. This can be useful for customer segmentation or identifying similar documents in text analysis.
- Association rules help you identify exciting relationships between items by analyzing transactional data. This can be valuable for market basket analysis, where you can determine which products are frequently purchased together.
- Anomaly detection helps identify unusual or suspicious behavior that may indicate fraudulent activities or system failures. For example, it can help detect credit card fraud by flagging transactions that deviate from standard spending patterns.
Explore different algorithms utilized in these techniques for practical analysis.
To implement these data mining techniques effectively, it is essential to understand the algorithms associated with each technique. Algorithms provide step-by-step instructions on how to perform the analysis and extract meaningful insights.
- Decision trees and Naive Bayes are commonly used classification algorithms that help build predictive models based on training data.
- K-means and hierarchical clustering algorithms are widely used for clustering tasks, allowing you to group similar objects.
- The Apriori algorithm is famous for association rule mining, helping identify frequent item sets and generate meaningful rules.
- Isolation Forest and Local Outlier Factor (LOF) are standard algorithms used for anomaly detection, enabling the identification of abnormal observations within a dataset.
Gain knowledge on selecting appropriate techniques based on specific business objectives.p
Choosing the correct data mining technique depends on your specific business objectives and the nature of your data. It’s essential to consider factors such as the type of data you have, the size of your dataset, and the goals you want to achieve.
For example:
- Classification techniques like decision trees or logistic regression may be suitable for predicting customer churn based on historical data.
- If you want to segment customers into different groups based on their purchasing behavior, clustering techniques like K-means or hierarchical clustering can be applied.
- Association rule mining using the Apriori algorithm would be appropriate.
Benefits and examples of data mining
Data mining is a powerful tool that can benefit organizations across various industries. By implementing a robust data mining strategy, businesses can gain valuable insights that drive decision-making, enhance customer targeting, detect fraud, and much more. Let’s dive in!
Improved Decision-Making
One of the key benefits of data mining is its ability to improve decision-making processes within an organization. By analyzing large datasets and extracting meaningful patterns and trends, businesses can make informed decisions based on accurate information rather than relying on intuition or guesswork.
- Data mining allows businesses to identify hidden patterns and correlations in their data, enabling them to uncover valuable insights that may not be apparent through traditional analysis methods.
- With these actionable insights, organizations can make more informed strategic decisions, optimize operational processes, and allocate resources effectively.
Enhanced Customer Targeting
Another significant advantage of data mining is its potential to enhance customer targeting efforts. By analyzing customer behavior patterns and preferences, businesses can tailor their marketing strategies to target specific segments with personalized offers and messages.
- Data mining enables businesses to segment their customer base effectively by identifying common characteristics or behaviors among different groups.
- This segmentation allows for targeted marketing campaigns that resonate with customers on a deeper level, leading to increased engagement and higher conversion rates.
- Data mining can help identify cross-selling or upselling opportunities by understanding customers’ purchasing habits and preferences.
Fraud Detection
Data mining plays a crucial role in detecting fraudulent activities within an organization. Businesses can identify suspicious patterns or anomalies that may indicate fraudulent behavior.
- Through advanced algorithms and machine learning techniques, data mining can flag unusual activities such as unauthorized access attempts or abnormal financial transactions.
- Detecting fraud early on can save businesses significant financial losses and protect their reputation.
- Examples of successful fraud detection through data mining include credit card companies identifying fraudulent transactions and insurance companies detecting false claims.
Successful Case Studies
To illustrate the power of data mining, let’s explore a few successful case studies where organizations have leveraged this technique for remarkable outcomes:
- Netflix: The popular streaming platform uses data mining to analyze user preferences, viewing habits, and ratings to provide personalized recommendations. This approach has significantly contributed to customer satisfaction and retention.
- Walmart: By analyzing customer purchase history and behavior patterns, Walmart utilizes data mining to optimize inventory management, improve supply chain efficiency, and predict demand accurately. This has resulted in substantial cost savings and enhanced customer experience.
- Amazon: With its sophisticated recommendation engine powered by data mining techniques, Amazon offers personalized product suggestions based on customers’ browsing history and purchase behavior. This strategy has contributed to increased sales and customer loyalty.
Identifying Opportunities within Your Industry
Now that we’ve explored the benefits of data mining and examined real-world examples, it’s essential to identify potential opportunities for applying these benefits within your industry or organization.
- Consider your business’s specific challenges or pain points and how data mining can help address them.
- Identify areas where you collect large amounts of structured or unstructured data that could be valuable for analysis.
- Explore how leveraging data mining techniques can lead to actionable insights that drive business growth or improve operational efficiency.
Data mining vs. data analytics and data warehousing
In the world of data, three critical disciplines play a crucial role in extracting insights and value from vast amounts of information: data mining, data analytics, and data warehousing. While these terms may sound similar, they have distinct focuses and purposes. Let’s look closely at each one to understand their differences and how they work together.
Differentiate between Data Mining, Data Analytics, and Data Warehousing concepts.
Data mining is the process of discovering patterns and relationships within large datasets. It involves using various techniques such as clustering, classification, regression, and association to uncover hidden insights that can be used for decision-making. On the other hand, data analytics focuses on extracting meaningful insights from data by applying statistical analysis and mathematical models. It aims to answer specific questions or solve problems based on available data.
Data warehousing, meanwhile, is the practice of storing and managing large datasets in a centralized repository called a data warehouse. This allows organizations to access and analyze their data efficiently. A data warehouse is a single source of truth that consolidates different types of data from various sources into one unified view.
Understand how data mining focuses on discovering patterns while data analytics emphasizes extracting insights from data.
Data mining specialists dive deep into datasets to identify recurring patterns or correlations that might not be immediately apparent. They use algorithms to sift through vast information and extract valuable nuggets to help businesses make informed decisions.
On the other hand, data scientists who specialize in analytics focus on understanding what those patterns mean for businesses or organizations. They apply statistical methods to interpret the findings from the mined dataset. Doing so gives them valuable insights that can drive strategic decision-making processes.
Explore the role of data warehousing in storing and managing large datasets for analysis purposes.
Data warehouses play a critical role in facilitating efficient analysis by providing a structured and organized environment for data storage. They are designed to handle large volumes of data, including structured, semi-structured, and unstructured data.
By consolidating different datasets into a single repository, data warehouses enable organizations to perform complex queries and analyses on vast amounts of information. This allows businesses to comprehensively view their operations, customers, and market trends.
Recognize the complementary nature of these three disciplines in leveraging data effectively.
While each discipline has its distinct focus, they are interrelated and complement each other in leveraging data effectively. Data mining helps identify patterns that may go unnoticed, while data analytics provides insights based on those patterns. These insights can then be used to make informed decisions or drive further analysis.
Data warehousing is the backbone of these processes by providing a centralized repository for storing and managing large datasets. It ensures that the necessary data is readily available for mining and analysis.
In today’s era of big data, where organizations have access to vast amounts of information from various sources such as social media, IoT devices, and streaming data, leveraging these three disciplines is crucial for staying competitive.
While there are distinct differences between data mining, data analytics, and data warehousing, they all play integral roles in extracting value from large datasets. Data mining focuses on discovering patterns; analytics extracts insights from those patterns, and warehousing provides a centralized repository for efficient storage and management. By leveraging these disciplines together, businesses can unlock the full potential of their data and make well-informed decisions.
Fundamental techniques and algorithms in data mining
In data mining, several basic techniques and algorithms form the foundation for extracting valuable insights from vast data. These techniques help uncover patterns, relationships, and trends that can drive informed decision-making. Let’s delve into some of these essential techniques and algorithms:
Decision Trees
Decision trees are a popular algorithm used in data mining to make decisions based on a series of if-then rules. They visually represent possible outcomes by splitting the data into branches based on different attributes or features. Each branch represents a decision or outcome, leading to further branches until reaching a final result.
Pros:
- Easy to understand and interpret.
- Suitable for both categorical and numerical data.
- Can handle missing values.
Cons:
- Prone to overfitting if not pruned properly.
- Limited ability to capture complex relationships.
Neural Networks
Neural networks mimic the structure and functionality of the human brain, consisting of interconnected nodes (neurons) organized in layers. Each neuron receives inputs, applies weights, processes them through an activation function, and produces an output. These networks excel at pattern recognition tasks such as image or speech recognition.
Pros:
- Ability to learn from large amounts of training data.
- Can handle complex relationships between variables.
- Robust against noise in the input data.
Cons:
- Requires significant computational resources for training.
- Interpretability can be challenging due to their black-box nature.
Genetic Algorithms
Genetic algorithms take inspiration from natural selection processes observed in biological evolution. They involve creating a population of potential solutions encoded as strings (chromosomes). The algorithm then applies genetic operators like mutation and crossover to produce new generations with improved fitness until an optimal solution is found.
Pros:
- Effective for optimization problems with many variables.
- Can handle non-linear relationships between variables.
- Provide global search capabilities.
Cons:
- Computationally expensive for large problem spaces.
- Convergence to an optimal solution is not guaranteed.
Outlier Analysis
Outliers are data points that significantly deviate from the norm or expected patterns. Outlier analysis techniques help identify and handle these anomalies in data mining. By detecting outliers, we can gain valuable insights into unusual events, errors, or fraud cases that may require special attention.
Pros:
- Can uncover hidden patterns and irregularities.
- Useful for anomaly detection in various domains.
- It helps improve data quality by identifying errors.
Cons:
- Determining the threshold for outlier detection can be subjective.
- Outliers may represent legitimate but rare occurrences.
These are just a few of the essential techniques and algorithms used in data mining. It’s important to note that each algorithm has strengths and limitations, making them suitable for specific data mining tasks. As a practitioner, understanding these nuances allows you to make informed choices when applying these techniques to your datasets.
Furthermore, it’s worth exploring advanced algorithms widely used in specialized areas such as text mining or image recognition. For example, text mining algorithms like Latent Dirichlet Allocation (LDA) help extract topics from extensive collections of documents, while image recognition algorithms like Convolutional Neural Networks (CNNs) excel at identifying objects within images.
Real-world use cases and industries utilizing data mining
Data mining is a powerful tool that finds applications in diverse industries, revolutionizing organizations’ operations. Let’s explore some successful use cases and industries extensively employing data mining.
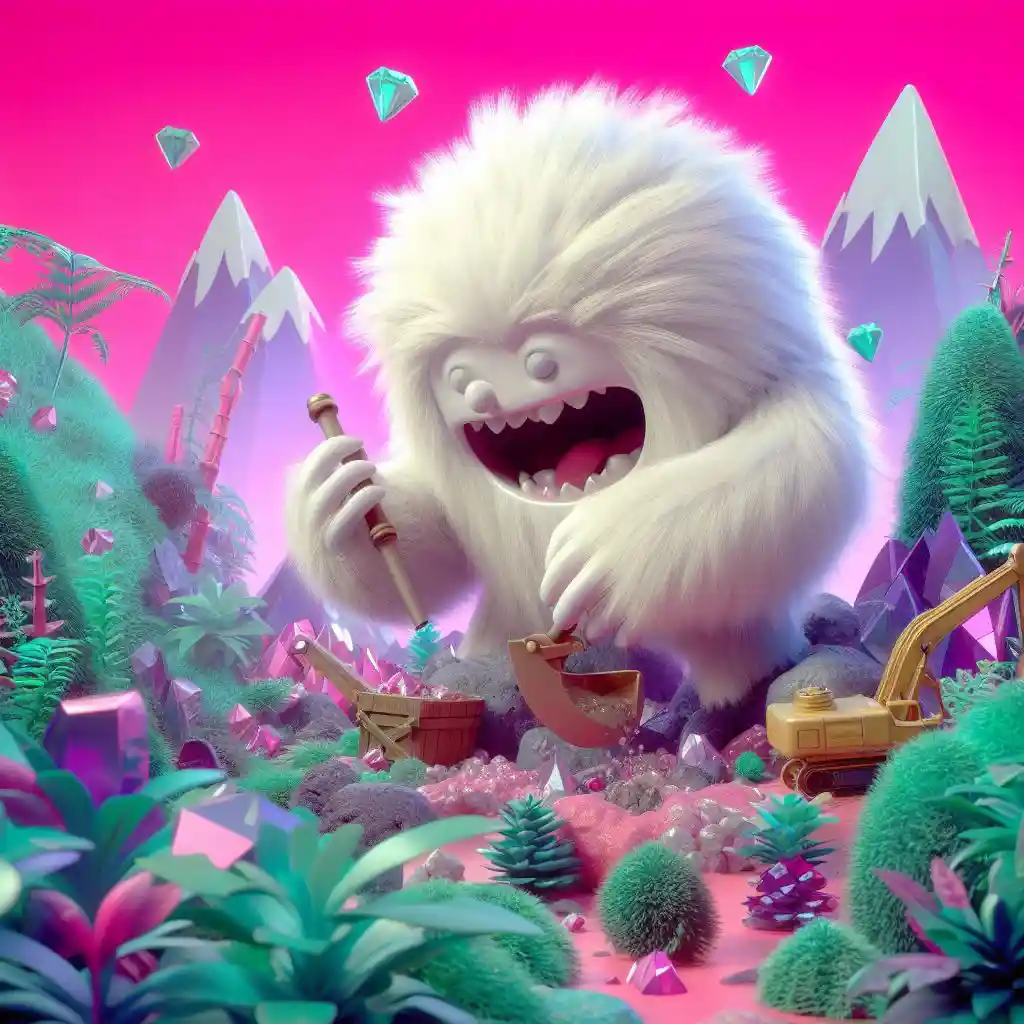
Discover diverse industries where data mining is extensively employed
Data mining has found its place in various sectors, from retail to finance to healthcare. In the retail industry, companies leverage data mining techniques to analyze customer purchasing patterns and preferences. By understanding these insights, businesses can tailor their marketing strategies and optimize product placement to maximize sales.
Data mining plays a crucial role in fraud detection in the finance sector. Financial institutions employ predictive modeling techniques to identify suspicious transactions or activities that deviate from normal behavior. This helps prevent fraudulent activities and protect customers’ financial assets.
The healthcare industry also benefits significantly from data mining. By analyzing patient records and medical histories, healthcare providers can identify trends and patterns that aid diagnosis and treatment planning. Data mining enables doctors to predict disease progression or potential complications accurately.
Learn about successful applications in retail, finance, healthcare, etc.
Let’s delve deeper into specific examples of how different industries utilize data mining:
- Retail: Companies like Amazon use data mining algorithms to recommend personalized products based on customers’ browsing history and purchase behavior. This strategy enhances the customer experience and boosts sales by increasing cross-selling opportunities.
- Finance: Credit card companies employ data mining techniques to detect real-time fraudulent transactions. By analyzing historical transactional patterns, they can identify unusual spending patterns or suspicious activities that indicate potential fraud attempts.
- Healthcare: Data mining facilitates early detection of diseases by identifying risk factors based on demographic information, medical history, lifestyle choices, genetic predisposition, etc. This proactive approach allows for timely interventions and improved patient outcomes.
- Manufacturing: By integrating real-time sensor data with historical production records, manufacturers can identify patterns that lead to equipment failure or production bottlenecks. This enables them to optimize maintenance schedules, reduce downtime, and improve operational efficiency.
Understand how organizations leverage data mining to optimize operations or gain a competitive edge.
Organizations across industries leverage data mining to optimize operations and gain a competitive edge. Here’s how:
- Improved decision-making: Data mining provides valuable insights that enable organizations to make informed decisions based on historical trends, patterns, and correlations. This helps identify market opportunities, streamline processes, and allocate resources effectively.
- Enhanced customer experience: By analyzing customer data, organizations can personalize their offerings, provide targeted recommendations, and deliver a seamless customer experience. This leads to increased customer satisfaction and loyalty.
- Cost reduction: Data mining helps identify business processes and supply chain inefficiencies. By optimizing these areas, organizations can reduce costs associated with inventory management, logistics, production planning, etc.
- Competitive advantage: Organizations utilizing data mining techniques gain a significant competitive advantage by staying ahead of market trends and consumer preferences. They can anticipate changes in demand patterns or emerging market segments and tailor their strategies accordingly.
Explore specific examples showcasing the impact of data mining on business outcomes.d
Data mining has had a profound impact on various organizations’ business outcomes. Let’s explore some specific examples:
- Netflix: The popular streaming platform leverages data mining algorithms to analyze user viewing habits and preferences. This enables them to recommend personalized content suggestions tailored to each viewer’s taste. As a result, Netflix has significantly improved user engagement and retention rates.
- Walmart: By analyzing sales data from millions of transactions daily, Walmart identifies buying patterns and adjusts its inventory accordingly. This ensures that popular items are always stocked while minimizing wastage due to overstocking or understocking.
Key takeaways from “Data Mining How To”
Lessons Learned in Data Mining
The “Data Mining How To” guide provides valuable insights into the world of data mining. By summarizing the lessons learned, we can better understand how to mine data and extract useful information effectively. Here are the key takeaways:
Concepts and Techniques in Data Mining
The guide covers various concepts and techniques related to data mining. These include:
- Data Points: Data mining involves analyzing significant data points to uncover patterns, trends, and correlations.
- Consumer Data: By mining consumer data, businesses can gain valuable insights into customer behavior, preferences, and future trends.
- Objectives: Defining clear objectives before embarking on a data mining project is crucial. This helps in focusing efforts toward specific business goals.
- Steps: The guide outlines step-by-step processes for successful data mining, including defining objectives, gathering and preprocessing raw data, applying algorithms, and interpreting results.
Practical Tips for Implementation in Data Mining
Implementing effective data mining strategies requires careful consideration of several factors. Here are some practical tips highlighted in the guide:
- Identify Relevant Data Sources: Gathering data from multiple sources that align with your business goals is essential to obtain accurate predictions and meaningful insights.
- Preprocess Raw Data: Before analysis can begin, raw data needs to be cleaned and transformed into a suitable format. This ensures accurate results during the mining process.
- Choose Appropriate Algorithms: Different algorithms serve different purposes in data mining. Selecting a suitable algorithm based on your objectives is critical for reliable results.
- Privacy Considerations: Privacy should be a top priority when working with users or personal data. Ensure compliance with relevant regulations and implement necessary security measures.
- Interpretation of Results: Data mining yields vast amounts of information; however, it is important to interpret these results correctly to make informed decisions for your business.
Further Exploration in Data Mining
While the guide covers essential concepts and techniques, data mining is a vast field with numerous advanced topics. Here are some areas worth delving into:
- Machine Learning: Understanding machine learning algorithms can enhance data mining capabilities by enabling more accurate predictions and uncovering complex patterns.
- Deep Learning: Exploring deep learning methodologies can provide insights into analyzing unstructured data, such as images, text, and videos.
- Big Data Analytics: As the volume of data continues to grow exponentially, exploring big data analytics can help businesses make sense of massive datasets efficiently.
By further exploring these advanced topics, you can stay ahead of the curve in the rapidly evolving field of data mining.
Relationship between Data Mining, Big Data, Machine Learning, and Artificial Intelligence
In analytics, there is a strong interconnectedness between data mining, big data, machine learning, and artificial intelligence. Let’s delve into how these fields are related and how they contribute to analyzing vast amounts of information.
Understanding the Interconnectedness
Data mining is a technique used to extract valuable insights from large datasets. It involves analyzing data patterns and relationships to uncover hidden information. Big data provides the raw material for data mining by offering massive amounts of structured and unstructured data that can be explored.
Machine learning algorithms play a crucial role in enhancing predictive capabilities within a dataset. Machine learning enables us to make accurate predictions or classifications based on new incoming data by training models on historical data patterns. These algorithms continuously learn from further information and improve their performance over time.
Artificial intelligence furthers this process by automating complex analytical tasks in analyzing large datasets. AI encompasses techniques like neural networks and deep learning algorithms that mimic human cognitive processes. These techniques enable machines to understand, reason, and decide based on the analyzed data.
Exploring Big Data’s Role in Data Mining
Big data serves as the foundation for effective data mining practices. With its vast volume, velocity, variety, and veracity, big data provides abundant information for analysis. Through techniques like data mining, we can sift through this immense amount of data to identify trends, patterns, correlations, and anomalies that might otherwise go unnoticed.
Data mining allows organizations to gain valuable insights into customer behavior, market trends, fraud detection, risk assessment, and more. By leveraging big data through advanced analytics techniques like clustering or association rule discovery in the context of retail sales transactions or web clickstream analysis, organizations can uncover hidden relationships among various entities or discover exciting patterns that drive business growth.
Enhancing Predictive Capabilities with Machine Learning
Machine learning algorithms are instrumental in enhancing the predictive capabilities of data mining. These algorithms can be trained to recognize patterns and make accurate predictions based on historical data. Machine learning models can learn to classify new instances or predict future outcomes by analyzing a dataset’s features and labels.
For example, in the healthcare industry, machine learning algorithms can analyze patient data to identify potential diseases or predict patient outcomes. This enables healthcare providers to make informed decisions about treatment plans and interventions.
Automating Complex Analytical Processes with Artificial Intelligence
Artificial intelligence automates complex analytical processes involved in analyzing large datasets. AI techniques like neural networks and deep learning models excel at recognizing intricate patterns and making sense of unstructured data such as images, text, or speech.
By leveraging artificial intelligence, organizations can automate tasks like sentiment analysis of customer reviews, image recognition for self-driving cars, or natural language processing for voice assistants. This automation saves time and improves efficiency and accuracy by reducing human error.
Data Mining and Knowledge Discovery in Databases (KDD)
In data science, knowledge discovery from databases (KDD) plays a vital role. KDD is not just about mining data; it encompasses several stages, including preprocessing, transformation, data mining, and interpretation. Let’s delve into how data mining fits into the broader picture of KDD.
Understanding the Significance of Data Mining in KDD
Data mining is a crucial step within the KDD process. It involves extracting valuable patterns or knowledge from large datasets to uncover hidden insights. By leveraging various techniques such as machine learning and statistical analysis, data mining allows us to identify meaningful relationships, trends, and patterns hidden within vast amounts of available data.
The Relationship between KDD and Other Disciplines
KDD shares a close relationship with other disciplines like machine learning and statistics. While machine learning focuses on developing algorithms that can learn from data automatically, statistics provides tools for analyzing and interpreting data. Data mining bridges these two fields by utilizing machine learning algorithms to discover patterns in large datasets.
Extracting Actionable Insights through Knowledge Discovery in Databases
The primary goal of database knowledge discovery is to extract actionable insights from raw data. With increasing information generated daily through various sources such as social media platforms, organizations have access to abundant valuable data. However, this wealth of information remains untapped without proper analysis and interpretation.
By applying techniques such as anomaly detection or network analysis during the KDD process, organizations can uncover valuable information that helps them make informed decisions. For example:
- Anomaly detection can help identify unusual behavior or outliers within a dataset.
- Network analysis can reveal complex relationships between entities, such as users or events in social media networks.
These insights enable organizations to optimize their operations, improve customer experience, detect fraud or security breaches, and gain a competitive edge in the market.
Ethical Considerations in Data Mining and KDD
While data mining and knowledge discovery offer immense potential for extracting valuable insights, it is essential to address ethical considerations. Organizations must have the consent or legal rights to collect and analyze data. Steps should be taken to protect individuals’ privacy and prevent any misuse of sensitive information.
Data scientists and analysts need to be aware of the ethical implications of their work and adhere to guidelines prioritizing transparency, fairness, and accountability. By incorporating ethical practices into the KDD process, organizations can build trust with their stakeholders while leveraging the power of data mining for positive outcomes.
Data Mining Software & Tools: A Comprehensive Overview
Having the right software and tools can make all the difference. With many options available, it is important to know which ones are popular, their features and capabilities, and their compatibility with different datasets. Whether you’re a seasoned data miner or just starting, selecting the right software or tool based on your project requirements is crucial. Let’s dive into this comprehensive overview of data mining software and tools.
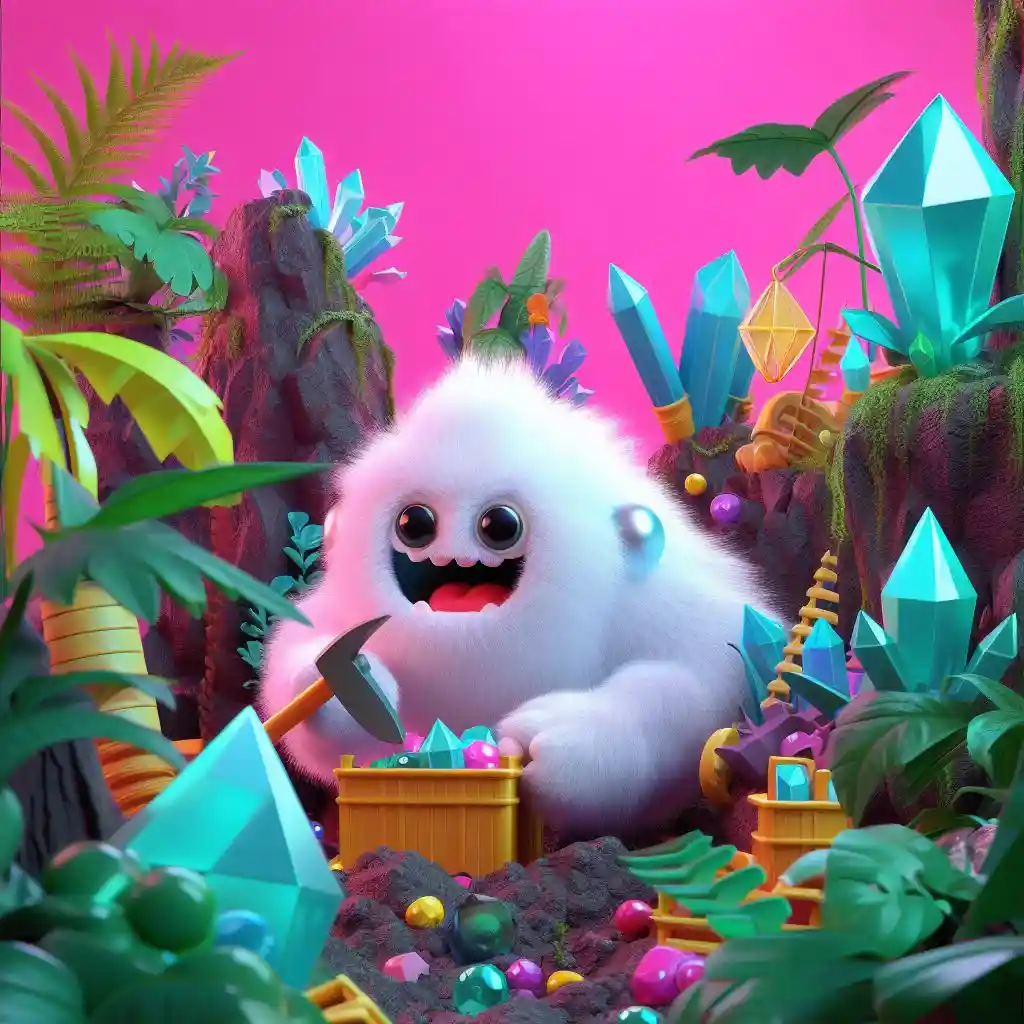
Discover Popular Software and Tools Used in Data Mining
Several options have gained popularity among data miners. These tools offer a wide range of functionalities to help extract valuable insights from large datasets. Some popular choices include:
- RapidMiner: First, This open-source tool provides an intuitive interface for beginners while offering advanced features for experienced users. It supports various data sources and algorithms, making it versatile for projects. It’s not as complex as Weka, the next bullet, but it looks technical.
- Weka: Second, Another open-source tool widely used in academia and industry, Weka offers a comprehensive suite of machine learning algorithms and preprocessing techniques. Its user-friendly interface makes it accessible to both novice and expert users. Weka is much more complicated than the next bullet.
- KNIME: Third, Known for its modular approach, KNIME allows users to build custom workflows by connecting pre-built nodes. It supports integration with other programming languages, such as R and Python, expanding its capabilities beyond traditional data mining tasks. We love Knime, the easiest of them; we offer Knime Consulting and have created a Knime Desktop Task Scheduler to schedule your KNIME workflows.
Learn About Their Features, Capabilities, and Compatibility
Every data mining software or tool has distinct features and capabilities tailored to address specific needs. Consequently, comprehending these aspects can help determine which option best aligns with your project requirements. To facilitate a more seamless understanding of these considerations, here are some key factors to keep in mind, along with transitional phrases:
First and foremost, regarding Data Preprocessing, it’s worth noting that many tools provide extensive preprocessing capabilities. These encompass cleaning noisy data, handling missing values, scaling variables, and transforming attributes. Transitioning to the next point,
Secondly, in Machine Learning Algorithms, diversity is crucial. It is imperative to have access to a wide range of machine-learning algorithms. Look for tools that offer a broad spectrum of algorithms, including decision trees, regression models, clustering techniques, and neural networks.
Moreover, in the context of data analysis and presentation, Visualization is of paramount importance. Ensuring that the software or tool you choose offers robust visualization capabilities is vital. This will enable you to explore and present your results effectively. Transitioning to the final consideration,
Lastly, when considering Compatibility, you should assess the software’s ability to work with different datasets and file formats. A good tool should support various data types, including structured, unstructured, time series, and text data.
Conclusively, we must begin considering all these aspects to help you decide which data mining software or tool is best suited for your specific project requirements.
Careers in Data Mining: Information Security Analyst and Market Research Analyst
So, you’re interested in data mining and want to explore potential career paths? Well, you’ve come to the right place! Let’s look at these roles, including the required skills, qualifications, and responsibilities.
Information Security Analyst
As an information security analyst, your primary focus will be protecting an organization’s data from unauthorized access or breaches. You’ll work closely with IT teams to identify vulnerabilities in systems and networks, implement security measures, and develop strategies to mitigate risks. Here are some key points about this career:
- Skills: Strong analytical skills are crucial for identifying potential threats and vulnerabilities. Knowledge of programming languages like Python or Java can be advantageous for analyzing data patterns.
- Qualifications: A bachelor’s degree in computer science or a related field is typically required for entry-level positions. Certifications such as Certified Information Systems Security Professional (CISSP) can also enhance your credentials.
- Responsibilities: Your primary responsibility as an information security analyst will be to monitor network activity for suspicious behavior or signs of intrusion. You’ll also conduct regular vulnerability assessments, create incident response plans, and educate employees on best practices for data protection.
Market Research Analyst
If you have a knack for numbers and enjoy uncovering consumer insights, a career as a market research analyst might be the perfect fit for you. Analysts gather and analyze data to help companies make informed business decisions. Here’s what you need to know about this role:
- Skills: Analytical skills are essential for interpreting market trends and consumer behavior. Proficiency in statistical software like SPSS or SAS is also valuable for analyzing large datasets.
- Qualifications: A bachelor’s degree in marketing, statistics, or a related field is typically required. Some employers may prefer candidates with a master’s degree in market research or a related discipline.
- Responsibilities: As a market research analyst, you’ll be responsible for designing and conducting surveys, analyzing data to identify market trends, and presenting findings to clients or internal stakeholders. You’ll also be involved in competitive analysis and forecasting future market conditions.
Job Prospects and Growth Opportunities
Now that we’ve explored the skills, qualifications, and responsibilities of information security analysts and market research analysts, let’s talk about the job prospects and growth opportunities in these fields:
- Information Security Analysts: With the increasing importance of data security, the demand for information security analysts is rising. According to the U.S. Bureau of Labor Statistics, employment in this field is projected to grow by 31% from 2019 to 2029, much faster than average.
- Market Research Analysts: The need for market research analysts grows as companies strive to understand consumer behavior and stay ahead of their competitors. The U.S. Bureau of Labor Statistics projects an 18% growth rate for this occupation from 2019 to 2029.
Both careers offer promising job prospects with competitive salaries. There are ample opportunities for career advancement and specialization within these fields.
Applications of Data Mining in Various Industries
Data mining has become invaluable in today’s data-driven world, revolutionizing how businesses operate across various industries. From e-commerce to telecommunications and manufacturing, organizations are leveraging data mining techniques to gain valuable insights and drive innovation.
E-Commerce
In e-commerce, data mining plays a crucial role in understanding customer behavior and optimizing marketing campaigns. By analyzing vast amounts of consumer data, businesses can identify patterns and trends that help them tailor their marketing efforts effectively. By examining purchase history and browsing habits, e-commerce companies can offer personalized product recommendations to customers, enhancing their shopping experience.
Telecommunications
Telecommunications is another industry that significantly benefits from data mining. With millions of subscribers generating massive amounts of call records and network data, telecom companies use data mining techniques to detect fraud, optimize network performance, and improve customer relations. They can proactively identify potential fraudulent activities or network bottlenecks by analyzing call patterns and network traffic.
Manufacturing
Data mining has also found its place in the manufacturing sector. Companies leverage mined data to optimize production processes, reduce costs, and improve product quality. By analyzing historical production data and identifying correlations between variables such as raw materials used or machine settings, manufacturers can uncover insights that lead to more efficient operations. This enables them to streamline workflows, minimize waste, and ultimately enhance productivity.
Healthcare
Data mining plays a vital role in improving patient care outcomes and reducing costs in the healthcare industry. By analyzing electronic health records (EHRs) or clinical trial results using advanced algorithms, healthcare providers can identify patterns that help predict disease progression or treatment effectiveness. This enables doctors to make more informed decisions about patient care while reducing unnecessary medical procedures or treatments.
Marketing
Data mining is a game-changer. Businesses can gain valuable insights into consumer preferences, allowing them to create targeted marketing campaigns and deliver personalized experiences. For instance, by segmenting customers based on their demographics or purchase history, marketers can tailor their messaging and offers to specific customer groups, increasing the likelihood of conversion.
Financial Services
The financial services industry heavily relies on data mining techniques for risk assessment, fraud detection, and customer relationship management. Banks and financial institutions can promptly identify potential fraudulent activities by analyzing transactional data and patterns in financial transactions. Data mining helps them assess creditworthiness accurately and develop customized financial products that meet individual customer needs.
Challenges Addressed by Data Mining
Each industry faces unique challenges that can be effectively addressed through data mining techniques. For example, businesses often struggle to understand complex consumer behavior or predict future market trends. Data mining provides the tools to quickly analyze vast amounts of data and extract meaningful insights that drive informed decision-making.
Moreover, organizations frequently encounter business problems that require a comprehensive understanding of their customers’ needs and preferences. By leveraging mined data, companies can better understand their target audience’s buying habits, enabling them to design products or services that align more closely with customer expectations.
History and Evolution of Data Mining: Tracing Its Origins
Data mining, a field that has revolutionized how we analyze and extract valuable insights from vast amounts of data, has a rich history dating back several decades.
Historical Development: Unearthing the Roots
The origins of data mining can be traced back to the mid-20th century when statisticians and researchers began exploring ways to extract meaningful patterns and information from large datasets. One of the earliest applications was agriculture, where statistical methods were employed to analyze crop yields and optimize farming practices. This laid the foundation for what would later become known as “data mining.“
Technological Advancements: Paving the Way for Progress
Advancements in computing power and storage capabilities in the 1970s and 1980s played a pivotal role in propelling data mining forward. With increased computational capacity, researchers could process larger datasets more efficiently, enabling them to uncover hidden patterns and relationships within the data. Introducing powerful algorithms, such as regression analysis, further enhanced their ability to make accurate predictions based on historical data.
Key Milestones: Shaping Current Practices
Over time, several key milestones have shaped the field of data mining into what it is today. Let’s take a closer look at some notable developments:
- Creation of Decision Trees: In the 1960s, decision trees emerged as an effective method for classifying data based on hierarchical decisions or rules. This approach paved the way for more sophisticated machine-learning algorithms widely used today.
- Introduction of Association Rules: In 1993, Agrawal et al. introduced association rules, a technique that allows for discovering relationships and associations between variables in large datasets. This breakthrough opened up new possibilities for market basket analysis and recommendation systems.
- Rise of Neural Networks: The resurgence of neural networks in the 1990s brought about significant advancements in data mining. These interconnected layers of artificial neurons can learn complex patterns and have been instrumental in various applications such as image recognition, natural language processing, and predictive modeling.
Influential Figures: Honoring Contributions
Throughout its history, data mining has been shaped by the contributions of visionary individuals who have pushed the boundaries of what is possible. Let’s highlight a few influential figures:
- John Tukey: Known as one of the pioneers in exploratory data analysis, Tukey made significant contributions to statistical techniques that laid the groundwork for modern data mining approaches.
- Leo Breiman: A prominent statistician and machine learning researcher, Breiman’s work on decision trees and random forests has profoundly impacted data mining algorithms.
- Jiawei Han: A leading figure in the field, Han has made significant contributions to association rule mining and pattern discovery, advancing our understanding of extracting valuable insights from large datasets.
Data Mining vs. Data Harvesting: Differentiating the Concepts
In the realms of data analysis and information gathering, two frequently encountered terms are “data mining” and “data harvesting.” Although they may sound somewhat similar, they have distinct purposes, methods, and ethical considerations, particularly when it comes to privacy. Gaining a clear understanding of these differences is essential for effectively applying these concepts and ensuring responsible and ethical data practices.
Data Mining
Purpose: Data mining primarily aims to uncover patterns, relationships, and insights within existing datasets. Its core purpose is to extract valuable knowledge from historical or existing data to inform decision-making, make predictions, and improve processes. Data mining often deals with large volumes of structured data and involves applying advanced analytical techniques to identify hidden patterns.
Methods: Data mining involves sophisticated analytical methods such as clustering, classification, regression analysis, association rule mining, and machine learning algorithms. It is a process of knowledge discovery from data, focusing on exploring and analyzing the existing data.
Ethical Considerations: Ethical considerations in data mining include issues related to data privacy, bias, and transparency. It’s essential to protect individuals’ privacy by anonymizing data and ensuring that the insights derived do not compromise sensitive information. Additionally, addressing bias in data and algorithms is crucial to prevent unfair outcomes in hiring or lending decisions.
Data Harvesting
Purpose: Data harvesting, in contrast, is primarily centered on collecting specific and targeted information from various sources, including websites, online databases, or APIs. Its core purpose is to acquire data immediately relevant to a specific need, such as lead generation, market research, or competitive analysis. Data harvesting focuses on gathering fresh data in real-time or near-real-time.
Methods: Data harvesting relies on automated tools, web scraping scripts, or APIs to extract data from online sources. It is a more straightforward process than data mining and doesn’t involve complex analytical techniques. The primary objective is efficient data collection.
Ethical Considerations: Ethical considerations in data harvesting primarily revolve around respecting the terms of service of websites and APIs. It’s crucial to ensure that data is collected legally and ethically and that web scraping or data extraction practices do not violate intellectual property or privacy laws. It’s essential to be transparent about data collection and usage and to obtain the necessary permissions.
In summary, while data mining and data harvesting involve data collection and analysis, they serve different purposes and use distinct methods. Data mining is focused on knowledge discovery from historical data, while data harvesting is more about acquiring targeted and real-time data for specific operational needs. Ethical considerations, especially concerning privacy and data usage, are essential in both practices, but the particular concerns may differ due to their distinct purposes and methods. Responsible data practices and compliance with relevant laws and regulations are critical to ensure ethical and legal data usage.
Define both concepts of data mining and data harvesting.
Data mining involves discovering patterns, correlations, or insights from large datasets. It consists in extracting valuable information from vast amounts of structured or unstructured data using various techniques such as machine learning algorithms, statistical analysis, and pattern recognition. Data mining aims to uncover hidden knowledge that can be used for decision-making or predictive modeling.
On the other hand, data harvesting involves collecting or gathering information from different sources for a specific purpose. It typically involves automated processes that extract data from websites, databases, social media platforms, or other digital sources. The collected data is stored for further analysis or utilized in various applications.
Highlight differences between them regarding purpose, methods used, etc.
Purpose
- Data Mining:
- Purpose: Data mining aims to discover patterns, relationships, and insights within existing, often large, datasets. It is a process of knowledge discovery from data.
- Use Cases: Data mining is used for various purposes, such as predicting customer behavior, optimizing business processes, improving healthcare outcomes, and more. Its primary goal is to extract valuable knowledge from historical data for decision-making and forecasting.
- Data Harvesting:
- Purpose: Data harvesting, on the other hand, is primarily focused on collecting specific and targeted information from various sources, including websites, databases, or APIs. It aims to acquire data relevant to a particular need, like lead generation or competitive analysis.
- Use Cases: Data harvesting is often used for market research, email marketing campaigns, competitive intelligence, and data enrichment. It is more about gathering fresh data for specific, immediate applications.
Methods Used
- Data Mining:
- Methods: Data mining employs advanced analytical techniques such as clustering, classification, regression analysis, association rule mining, and machine learning algorithms. It involves exploring and analyzing existing data to uncover hidden patterns and relationships.
- Data Source: Data mining typically works with structured data from databases and other sources.
- Data Harvesting:
- Methods: Data harvesting relies on automated tools, web scraping scripts, or APIs to extract data from online sources. It doesn’t involve complex analytical techniques but rather focuses on efficient data collection.
- Data Source: Data harvesting mainly targets unstructured or semi-structured data from websites, social media, or online databases.
Scope
- Data Mining:
- Scope: Data mining analyzes historical data to identify trends, patterns, and insights. It often works with a large dataset that contains a wealth of information.
- Time Frame: Data mining can provide insights into long-term historical data, helping organizations make strategic decisions.
- Data Harvesting:
- Scope: Data harvesting involves acquiring specific, real-time, or near-real-time data relevant to a particular task or project. It has a more limited scope compared to data mining.
- Time Frame: Data harvesting is focused on the present or recent data, and its results are typically used for immediate, tactical purposes.
Application
- Data Mining:
- Application: Data mining finds applications in diverse fields such as finance (credit risk assessment), healthcare (disease prediction), marketing (customer segmentation), fraud detection, and recommendation systems. It is used for strategic decision-making and long-term planning.
- Data Harvesting:
- Application: Data harvesting is commonly used for more specific and short-term applications, like gathering leads for a sales campaign, monitoring competitor prices, or collecting data for a research project. It is geared toward operational and tactical activities.
In summary, data mining is a broader and more analytical process aimed at discovering valuable insights from historical data, while data harvesting is a more targeted and operational process focused on collecting specific information from online sources for immediate use. Both have their unique roles and applications in the world of data-driven decision-making.
Emphasize ethical considerations surrounding privacy when collecting or analyzing personal information.w
Ethical considerations are paramount. Privacy concerns arise due to the potential collection or analysis of personal information without consent. Handling data responsibly and adhering to legal regulations such as GDPR (General Data Protection Regulation) or CCPA (California Consumer Privacy Act) is crucial. Here are some key points to consider:
- Consent: Ensure individuals know how their data will be collected and used. Obtain explicit consent whenever necessary.
- Anonymization: Remove personally identifiable information from datasets to protect individuals’ privacy.
- Data Security: Implement robust security measures to safeguard collected data from unauthorized access or breaches.
- Transparency: Communicate the purpose of data collection and provide individuals access to their data.
Clarify distinctions to ensure accurate understanding and application of these terms.
To avoid confusion between data mining and data harvesting, it’s essential to clarify their distinctions:
- Purpose: Data mining focuses on discovering patterns within existing datasets for insights and decision-making. Data harvesting involves collecting specific information from various sources for a particular purpose.
- Methods Used: Data mining employs advanced analytical techniques like machine learning algorithms, while data harvesting utilizes automated tools or scripts for web scraping or targeted extraction.
- Scope: Data mining deals with large-scale analysis of existing datasets, while data harvesting involves actively gathering new information from online sources.
By understanding these differences, practitioners can apply the concepts appropriately while ensuring responsible use of collected information.
FAQs in Data Mining
What are some popular data mining tools?
There are several popular data mining tools available in the market today. Some widely used ones include RapidMiner, KNIME, Weka, Python libraries like sci-kit-learn and TensorFlow, and SQL-based platforms such as Oracle Data Mining and IBM SPSS Modeler.
Can data mining be applied to small businesses?
Absolutely! Data mining is not limited to large corporations; it can benefit small businesses too. By analyzing customer behavior patterns, identifying market trends, or optimizing inventory management, small businesses can make informed decisions that lead to growth and success.
Is data mining only valid for specific industries?
Data mining has applications across various industries,r including finance, healthcare, retail, marketing, telecommunications, and more. Its versatility lies in its ability to extract insights from diverse datasets regardless of industry type.
What skills are essential for a career in data mining?
To excel in data mining careers, having a solid foundation in statistics and programming languages like Python or R is crucial. Skills such as problem-solving abilities, critical thinking skills, and domain knowledge are highly valued in this field.
How can I get started with data mining?
To start with data mining, you can begin by learning the basics of statistics and programming languages like Python or R. Online courses and tutorials provide hands-on experience with popular data mining tools. Practice on real-world datasets and explore different techniques to gain practical knowledge in this field.
Remember, data mining is a journey of exploration and discovery. Embrace the process, continuously learn, and stay curious about the insights waiting to be unearthed from the vast realm of data!
Conclusion: Key Takeaways from “Data Mining How To”
Congratulations on completing the journey through our blog post, “Data Mining How To”! We’ve covered a wide range of topics related to data mining, from its concepts and techniques to real-world applications and career opportunities. Now that you have a solid understanding of the fundamentals, it’s time to implement your knowledge.
Data mining is like being an explorer in a vast jungle of information. Armed with the right tools and techniques, you can uncover valuable insights that will guide decision-making and drive innovation. So don’t hesitate to dive deep into the world of data mining and start extracting those hidden gems waiting to be discovered!