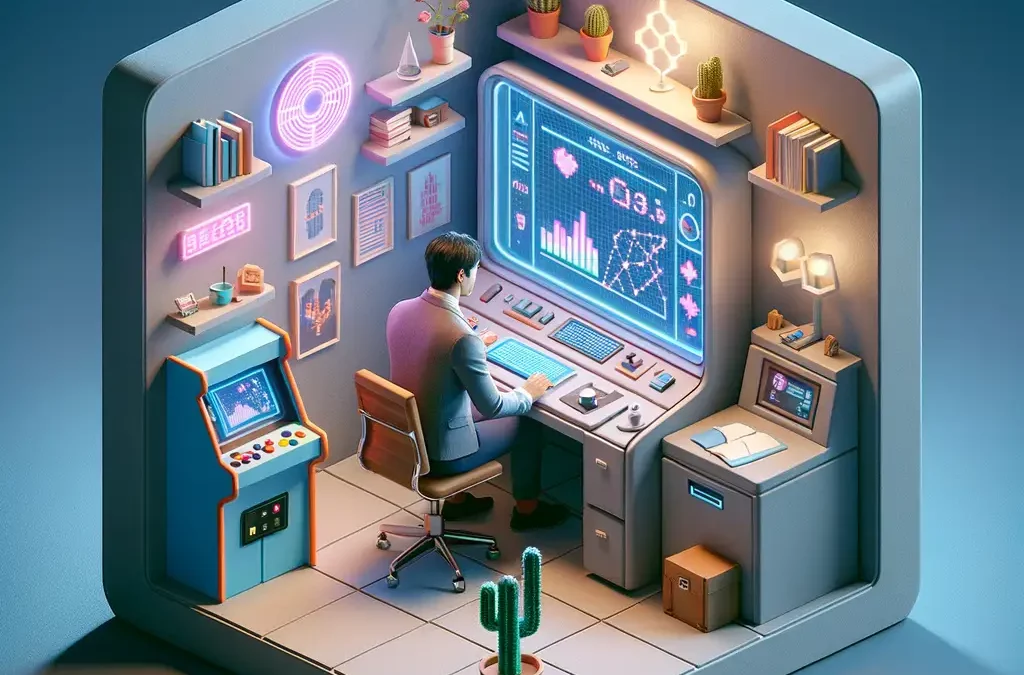
When to Use a Data Lake vs. a Data Warehouse
In today’s data-driven world, businesses are swimming in an enormous sea of information. Decision-makers seeking to harness the power of data must navigate a vital consideration: when to use a data lake versus a data warehouse. Choosing the correct architecture isn’t just about technology—it’s a strategic decision impacting analytics agility, innovation, and business insights. As advisors at the forefront of data engineering consulting services, we’ve observed the powerful impact the right storage and processing environments can have on organizational success. Let’s explore the distinctions between data lakes and data warehouses, and offer strategic insights on determining the best option to empower your analytics and accelerate your path to innovation.
What is a Data Lake?
A data lake is essentially a centralized repository that allows organizations to store vast amounts of data—structured, semi-structured, and unstructured—in its native format. Imagine it as a vast digital reservoir designed to handle the sheer scale and variety of modern data, from images and documents to logs, IoT sensor data, and beyond. Unlike traditional databases, data lakes defer schema and structure decisions until the moment of analysis, giving your analysts and data scientists ultimate flexibility. This flexibility accelerates innovation, allowing them to explore hypotheses without being hindered by rigid data schemas upfront.
Because of its flexibility, a data lake often serves as the foundational layer for machine learning projects, exploratory analytics, and big-data oriented tasks. For instance, businesses dealing with extensive log data or real-time data streams may prefer data lakes to capture diverse datasets efficiently, enabling them to extract insights or apply predictive modeling solutions quickly. Data lakes leverage technologies like Apache Hadoop, Amazon S3, or Azure Data Lake, optimized to handle the scale and complexity associated with massive datasets.
Choosing a data lake signifies adopting a methodological shift toward agile analytics and data science experimentation. For sophisticated organizations that understand the complexities and potential of modern analytics ecosystems and are comfortable orchestrating robust data engineering consulting services, a data lake becomes invaluable for powering agile methodologies and experimentation.
What is a Data Warehouse?
A data warehouse is a structured, predefined repository, optimized specifically for structured data analytics and reporting. Unlike the more flexible data lake that accepts data in raw formats, data warehouses enforce a schema upon ingestion. This structured data format makes them ideal for operational decision-making, business intelligence tasks, and traditional analytics. Data warehouses, equipped with a powerful operational schema and meaningful business rules defined at ingestion—unlike a data lake—offer business users quick access to accurate, reliable data for analysis and reporting.
Data warehouses leverage SQL-friendly technologies such as Amazon Redshift, Snowflake, or Google BigQuery. They allow analysts and executives to configure dashboards, visualize business KPIs rapidly, and empower organizations to make informed strategic decisions quickly and cost-effectively. If your enterprise needs accurate, consistent reporting and business-focused analytics, leveraging a data warehouse can accelerate daily analytic productivity exponentially.
Moreover, high-quality semantic modeling becomes more manageable when using a data warehouse infrastructure. Building clear data definitions and applying business context to your datasets through a robust semantic layer greatly enhances your teams’ ability to communicate and understand data clearly. A semantic layer built on top of a data warehouse also helps improve collaboration between your analytics teams and business stakeholders, ultimately leading to more actionable insights or answering pressing questions efficiently.
Key Differences: Comparing Data Lakes and Data Warehouses
Data Structure and Storage Approach
The most fundamental difference between data lakes and data warehouses lies in their respective approaches to data structure. Data lakes store raw data sets in their native format, ready for any future analytics scenario. Functionalities like flexible schema-on-read allow data scientists to define structure only when accessing data, which can be perfect for experimentation and agile analytics. Conversely, data warehouses follow the schema-on-write approach, requiring structure upfront to facilitate quicker queries and ensure data consistency and quality. This means warehousing takes more initial setup, but analytics later becomes more streamlined and efficient.
Types of Users and Analytic Workloads
A data lake typically caters to technical users like data scientists, technical analysts, and machine learning engineers who prefer to explore and experiment. They thrive on flexibility and the ability to leverage raw and diverse data quickly and autonomously. Data warehouses, in contrast, typically support business analysts, decision-makers, and non-technical users who seek consistent and secure access to structured data reports, dashboards, and well-defined insights. Knowing who your primary stakeholders are and analyzing different analytic workloads will provide clarity and strategic understanding of which solution aligns seamlessly with your organization’s current and long-term needs.
Performance and Scalability Considerations
Data lakes offer horizontal scalability designed to manage substantial data volumes and variety with ease. But analyzing these massive, diverse datasets could be slower when compared with data warehouses because of the on-demand structuring process at query-time. On the other hand, data warehouses, optimized for speed and predictable query performance, deliver faster analytical query responses by facilitating indexing and structured storage upfront. Considering these differences in performance and scalability allows your organization to choose the proper approach depending on the priority of speed, analytics agility, and scalability needs.
When to Choose a Data Lake vs. a Data Warehouse?
Deciding between a data lake and data warehouse should always align closely with your organization’s current and projected data strategy, goals, and culture. Choose a data lake formula if your priority involves agile analytics, machine learning capabilities, or experimental quick access to varied and evolving datasets. It’s ideal for industries generating massive volumes of highly variable data types—such as IoT, manufacturing, healthcare, or e-commerce.
Conversely, a data warehouse proves invaluable when timely, secure reporting and analytics access are your top priority. If structured data, accuracy, and consistency define your analytics strategy—especially within traditionally data-intensive fields like financial services, retail transactions, and operational reporting—then data warehouses will deliver immediate value and ease of use. Additionally, organizations taking steady first steps into analytics often find warehouses a more accessible starting point due to their inherent structure and usability.
Experienced practitioners often recommend a hybrid approach, delivering the best of both worlds: combine a data lake to handle flexibility, broad access, machine learning, and experimentation needs with a complementary data warehouse for reliable, consistent, and efficient operational analytics and reporting.
Integrating Analytics Strategies Successfully
Navigating the data lake versus data warehouse waters may seem challenging, but experienced guidance ensures you maximize the benefits of your analytics efforts. Effective data strategies mean more than merely picking a solution; it means understanding your business objectives, operational priorities, and teams’ capabilities. If your organization faces challenges with data miscommunication during analytics projects, carefully organized data engineering consulting services and targeted semantic layer implementations become crucial for achieving clarity, alignment, and innovation.
Whether engaging in agile experimentation within a data lake setup, implementing rapid single-processor workflows through Node.js asynchronous processing, or crafting structured, reliable insights through a warehouse approach—the most successful pathway forward is marrying analytics innovation with practical and actionable data strategies tailored to your specific goals and industry demands.
Remember, your organization’s analytics journey begins with aligning strategic considerations, team capabilities, and business objectives—choosing correctly between a data lake or data warehouse becomes transformational. Ready to innovate and explore what works best for you? Start your analytics transformation journey today.