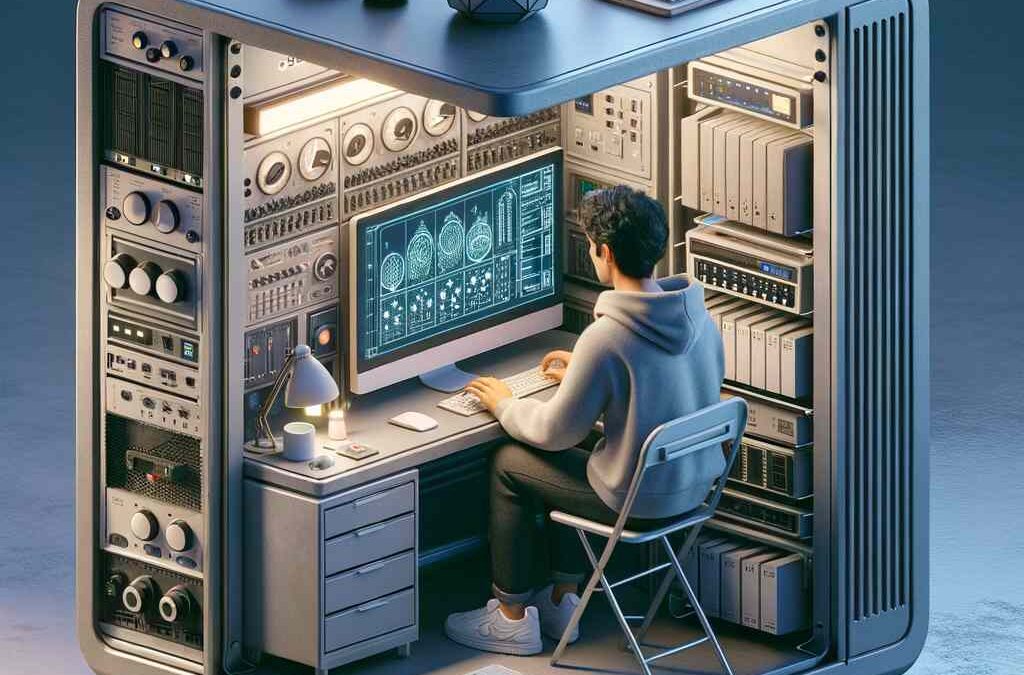
Data Pipeline Circuit Breaker Pattern Implementation
In an era where data pipelines fuel crucial business decisions, even brief disruptions can lead to massive operational and financial consequences. Imagine your organization’s revenue forecast suddenly stalls due to unforeseen data pipeline failure, creating blind spots in decision-making and analytics paralysis. To address these challenges proactively, leading data-driven companies are adopting the circuit breaker pattern—a proven strategy from software engineering applied innovatively to data pipelines. By strategically implementing a circuit breaker pattern, organizations can enhance their data operations resilience, reduce downtime, and maintain accurate insights when it matters most. For leaders ready to innovate their data strategy even further, engaging with knowledgeable teams providing expert guidance like our Power BI consulting services can turn these disruptions into opportunities for growth.
Understanding the Circuit Breaker Pattern
Widely recognized for its efficiency in traditional software development, the circuit breaker pattern is an architectural pattern designed to add resiliency by preventing cascading failures in complex information systems. Picture it as an electrical circuit breaker in your home—detecting abnormalities, stopping the flow, and preventing larger-scale impacts. Similarly, when applied to data pipelines, this pattern acts as a safeguard, proactively identifying and containing issues before they ripple through the system.
Specifically, the circuit breaker pattern monitors pipeline services and continuously checks their health and responsiveness. Whenever a dependency becomes unresponsive or sluggish, the circuit breaker reacts by opening the circuit to halt data flow, isolating the issue. Relevant teams receive immediate alerts, enabling rapid troubleshooting, targeted resource allocation, and quicker resolution. This containment strategy avoids overwhelmed processing components, which could otherwise degrade entire data platforms. To implement this effectively, it’s critical for data engineers—distinct from data analysts as explained in our detailed post on the differences between a data engineer and a data analyst—to clearly understand performance metrics and establish optimal thresholds for triggering.
Why Data-Driven Organizations Need Circuit Breakers
Data pipelines often span integrated ecosystems with numerous services operating continuously, extracting, transforming, loading, and visualizing substantial amounts of data in real-time. Any disruption creates immediate blind spots, misaligned forecasting, reduced operational intelligence, and diminished clarity in business decisions. Companies regularly generating demand prediction, a topic we discuss in depth in our article about accurate demand prediction for business success, need absolutely reliable pipelines. Interruptions or delays in accessibility to timely data often translate directly into lost revenue opportunities and competitive disadvantages.
Integrating a circuit breaker pattern into data pipelines dramatically enhances system resilience. Rather than allowing defective processes and unresponsive services to cascade across the entire data ecosystem, organizations leverage systematic circuit breaks to isolate and quickly resolve issues without extended downtime. Think about ambient data governance, embedding quality control directly into pipelines; we cover such methods comprehensively via our guide on ambient data governance for quality control within pipelines. Implementing the circuit breaker strategy aligns directly with these quality-focused principles and ensures that data remains consistent, timely, accurate, and actionable.
Planning Your Circuit Breaker Integration Strategy
Implementing a circuit breaker pattern requires strategic planning, careful execution, and continuous iteration informed by data-driven insights. Begin by enumerating your data pipeline components and dependencies, classifying them to identify service-critical units. Prioritize circuit breaker deployment across these critical but failure-prone areas to maximize resilience. Importantly, connect thresholds for circuit breaker opening directly to stressing parameters like response latency, error rates, and service uptime.
Organizations possess varied analytical budgets and resources, demanding careful prioritization of analytics projects; we discuss detailed strategies for balancing these considerations in our guide on prioritizing analytics projects with limited budgets. From this strategic starting point, organizations must select use cases wisely. Pilot implementations leveraging low-risk, yet impactful pipelines deliver valuable insights guiding the broader implementation initiative.
During planning, clearly differentiate roles and responsibilities between your data team members—ensuring clearly communicated responsibilities between technicians and analysts. For deeper insights into evolving talent pipelines, consider exploring our insights into the role of the University of Texas at Austin in training data analysts, which highlights how to invest in staff skills aligning with the modern data landscape.
Best Practices for Realizing Circuit Breaker Benefits
For optimal performance, incorporation of certain best practices has been crucial to organizations that successfully harnessed circuit breaker advantages. Organizations experience significantly boosted reliability when clearly defined state transitions (open, half-open, closed states) become integrated into pipeline monitoring. Once a data pipeline system tracers circuit breakers, real-time logging should be accessible through centralized dashboards and enhanced through comprehensive analytics. Ensure understanding of SQL usage across data operations is clear, employing our helpful article Demystifying the FROM clause in SQL: Understanding table selection and joining to educate teams effectively.
To maintain data accuracy, consider adding SQL views for dynamic status management to reflect circuit state changes efficiently and flexibly within relational databases. Our step-by-step SQL tutorial, CREATE VIEW: Creating virtual tables with query results in SQL, can guide teams effectively on creating actionable views.
Furthermore, regularly training personnel ensures continued alignment with underlying tech applications. Logical use of relational database principles helps sustain favorable data quality and rapid processing performance; refer further to our article on maximizing data processing speeds through relational theory and normalization which explains how to structure datasets effectively.
Visualizing Circuit Breaker Performance for Maximum Impact
Visibility matters—especially for non-technical stakeholders relying directly on timely data availability. Complementing technical implementation, leverage interactive dashboards and real-time alert notifications designed through best practices in data visualization—precisely detailed in our comprehensive article on Data Visualization Best Practices: A Quick Guide.
Employ interactive data visualization techniques from that guide to present practical insights clearly, enabling immediate drill-down on circuit breaker status, outage incidents, pipeline latency, and overall quality metrics. Dashboards need clarity, summarizing complex situations into easily digestible visuals. Achieving this effect demands intuitive communication, leveraging visual narratives to promptly alert stakeholders of potential pipeline impacts associated with circuit breaker states and shifting business-critical data availability.
An accessible dashboard, combined with automated alert emails, SMS, or chat-based notifications, raises immediate awareness, activating teams swiftly during incidents. These initiatives efficiently blend technology and user-centric design principles, maximizing the value of the implemented circuit breaker pattern and empowering decision-makers across the organization.
Driving Innovation with Proactive Pipeline Management
Data Pipeline Circuit Breakers represent an innovation benchmark for organizations seeking agility, robustness, and competitive advantage in today’s fickle digital environments. Ensuring reliable access to high-quality analytics is not merely a technical requirement—it’s fundamentally strategic. By proactively embedding circuit breaker patterns, engineering teams and business strategists collaboratively transform vulnerability points into new data innovation opportunities.
Enabling such strategic mastery is integral to our vision as technology consultants versed deeply in data analysis, visualization techniques, and challenging systems management. From establishing user-centric visualization platforms to fine-tuning analytics strategies with our established Power BI consulting services, we help businesses turn data into true-level competitive advantages. Choosing proactive pipeline safeguards through the circuit breaker pattern true empowers your teams to deliver unwavering business insights reliably, strategically advancing toward data-driven futures.
Tags: data pipeline architecture, circuit breaker pattern, data pipeline reliability, data engineering best practices, data analytics strategy, proactive data governance