Picture this: your team just discovered key customer insights, unlocked hidden market opportunities, and significantly shortened your decision cycle—all thanks to accurately handling data at lightning speed. This isn’t some future scenario; it’s the rapidly approaching reality of successful data-driven organizations in 2025.
As volumes of enterprise-level data grow exponentially, traditional ETL (Extract, Transform, Load) processes are increasingly struggling to keep pace. Enter ELT (Extract, Load, Transform)—a modern data management approach reshaping the way businesses optimize efficiency and insights from their data resources.
If your organization aims to remain competitive and proactive in the evolving technological landscape, mastering ELT strategies will be critical to your success. In this article, we’ll explain precisely why ELT is becoming the standard solution ahead of ETL by 2025, dissecting the underlying reasons why forward-thinking enterprises already embrace this shift.
Traditional ETL Processes: Why They’re Showing Their Age
Since the inception of data warehousing, ETL has long stood as the default method businesses use to move data from diverse sources into a centralized warehouse. While ETL processes were revolutionary in their time, efficiently structuring enormous amounts of information, they’re now beginning to reveal their inherent drawbacks. Primarily, ETL architectures were designed in an era when storage was expensive, connectivity was slower, and computing power was limited. This required businesses to carefully select, extensively clean, and meticulously transform data before loading it into costly warehouse environments. Consequently, ETL processes are inherently resource-intensive, complex, expensive to maintain, and challenging to scale across large datasets. Additionally, ETL workflows typically demand significant upfront planning in data modeling, forcing businesses into cumbersome processes before gaining valuable insights.
Today, business agility hinges on quick and informed decision-making capabilities. Unfortunately, traditional ETL architectures are not built to handle these modern demands effectively. As the sophistication of analytics increases, the depth and complexity of your datasets requires flexibility that older ETL methods simply cannot match. For a deeper understanding into ETL tool selection struggles businesses often face, check out our detailed article: How to choose the right ETL tool for your business.
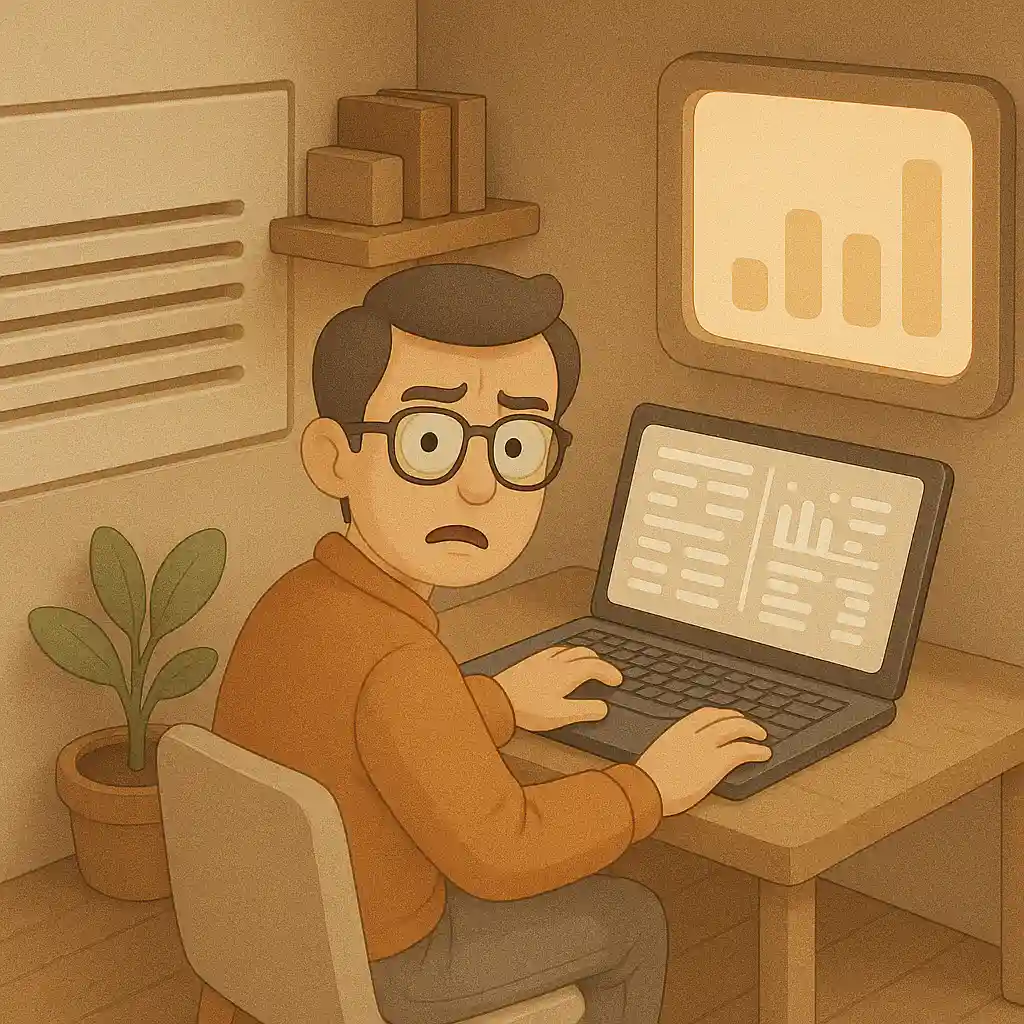
The Rise of Cloud-Based Infrastructure: Accelerating the ELT Transition
Cloud computing transformed the landscape of data storage and processing capabilities. Cloud-based infrastructure options such as AWS, Azure, Google Cloud, and Snowflake have altered the economics fundamentally: storage is affordable, scalability is flexible, and computing resources can be provisioned instantly. This shift towards affordable cloud resources significantly reduces the necessity of performing complex data transformations prior to the loading process, one of the fundamental principles behind classic ETL.
Enabled by this rapid growth in cloud capabilities, ELT processes reverse the order by first extracting and loading data into an enterprise’s data warehouse or data lake, leaving transformations to occur later within the powerful storage and computational environment. With ELT, organizations embrace agility by streamlining integration cycles, adapting more rapidly to changes in business requirements, and reducing infrastructure complexity. For organizations evaluating their current infrastructure, consider exploring our strategies for data warehouse adoption: 5 Signs your business needs a data warehouse today. ELT capitalizes on modern solutions like these, making it significantly more logical for resource-intensive analytics and predictive modeling in 2025.
Enhanced Speed and Agility in Analytics and Business Intelligence
One of the biggest competitive advantages ELT offers is its speed-to-insights. Traditional ETL processes are highly linear and require extensive upfront transformation steps. Delays and resource constraints frequently hinder organizations from quickly accessing critical data. ELT streamlines data extraction and loading processes, significantly shrinking the time-to-value gap. Once integrated into cloud-native databases such as Redshift, BigQuery, or Snowflake, data transformations happen efficiently within these robust, scalable platforms. Organizations can more rapidly deploy sophisticated analytics approaches, such as predictive modeling and machine learning, enhancing decision-making capabilities at unprecedented speeds.
Rather than limiting innovation to data modeling confines predetermined by ETL, businesses employing ELT benefit from greater agility. Data Scientists and business analysts gain access to raw data much earlier, empowering them to uncover innovative insights organically, without legacy process constraints. Real-time presence indicators or instant customer analytics initiatives, for example, require rapid data access and processing. Learn more about improving application responsiveness with advanced data capabilities in our article: Real-time presence indicators to improve apps.
Supporting Advanced Analytics and Predictive Models
Data-driven decision-making has evolved from a mere competitive advantage into an operational necessity by 2025. Real-time and predictive analytics, artificial intelligence, and machine learning initiatives require rapid access to diverse datasets in a timely manner. While ETL traditionally limits analysts with pre-modeled, heavily structured data, ELT provides direct, unfettered access to raw data. Without early-stage bottleneck limitations, your data teams possess greater freedom and flexibility, enabling rapid experimentation and iteration of advanced analytical models.
When your organization trusts real-time decisions and predictive modeling directly tied to business strategy, reliable, granular, and timely data becomes critically important. Check out our client-focused data journey where businesses shifted strongly from intuition-driven approaches to data-backed predictive modeling: From gut feelings to predictive models: a client journey. ELT solutions better align with these new analytics demands, accommodating explorative analytics and innovative modeling practices effortlessly.
Democratizing Data and Encouraging Collaboration Across Teams
ELT contributes significantly to making data analytics available organization-wide. Through ELT’s simplified structure, data analysts, business users, and technical specialists alike can access essential business insights without cumbersome waiting periods or heavy technical barriers. The simplicity, scalability, and efficiency offered by ELT architectures break down walls between internal departments, promoting collaboration across teams and creating clear avenues for data-driven conversations.
Shifting toward ELT also supports effective data visualization practices crucial in making data accessible to non-technical team members. Visualization tools can directly tap into large datasets more efficiently via cloud-native platforms, enabling dynamic, self-service BI dashboards and insights. For practical visualization optimization guidance, refer to our extensive guide: Data visualization best practices: a quick guide. By nurturing collaboration and a data-driven culture through ELT, businesses increasingly gain relevant and timely insights across critical initiatives including public safety, agile innovation, customer experience, and more. Specific examples like our Austin public safety data analytics case highlight these cross-function collaborative advantages effectively: The role of data analytics in enhancing public safety in Austin.
Future-Proofing Your Data Strategy with ELT
As the digital landscape expands exponentially, data modeling must evolve alongside it. To successfully accommodate future demands, ELT methodology inherently encourages flexible and adaptable data modeling practices, moving organizations toward sustainable success rather than rigid structures prone to becoming outdated quickly. Check out our discussion on modeling practices and their critical role in modern data strategy: Why data modeling is the blueprint for data-driven success.
Adopting ELT in 2025 safeguards your data strategy against constant industry evolution, growing data demands, and increasing competition. From rapid innovation support, advanced analytics compatibility, improved collaboration, and unprecedented agility in decision-making processes—eliminating legacy ETL limitations becomes logical and necessary if your business plans to thrive in the data-driven, hyper-connected world of 2025 and beyond.
Considering implementation? Our expert data consultants specializing in modern data architecture, data analytics, and MySQL databases can assist your organization in seamlessly transitioning to an ELT-driven strategy. Visit our MySQL Consulting Services to discover how we help bring your data initiatives to life.