Data Scientists often don’t know SQL and get stuck fixing excel based analytics in many cases. Gaining the degree doesn’t mean you leave knowing about relational theory.
With that said, when businesses first consider expanding their data capabilities, “data scientist” is often the first role to come to mind but it’s the last one on our mind.
Data scientists have become celebrities in the realm of data analytics, hailed as visionaries equipped to conjure predictive magic from endless streams of unstructured data.
Yet, though their advanced skills in machine learning and statistical modeling are powerful, hiring a data scientist as your first data team is often a huge mistep.
Before taking that crucial leap in data warehousing and data engineering, data science folk will not have a lot of data science to complete. Data engineering and data warehousing is vital but not exactly a skill for a data science guru.
The Real Mission Is Structuring Your Data First
Hiring a data scientist early can feel like buying a race car before you’ve even built your racetrack. For data scientists to deliver meaningful insights, they require well-structured, well-organized data that is easy to access, query, integrate, and analyze. Most organizations initially have disparate systems, messy data silos, spreadsheets hosted locally, and scattered analytics processes. Before any advanced analytics can truly be applied, someone needs to organize these data sets efficiently—this usually means investing in a skilled data engineer or analyst first.
Understanding your organizational data means building robust pipelines and data integration workflows to transform your raw data into clear, well-governed formats. Establishing a strong foundation with solid data warehouse architecture is crucial. In fact, there are clear signs your business needs a data warehouse today that shouldn’t be overlooked. Investing in proper structuring upfront streamlines all future analytic endeavors, simplifying the eventual introduction of sophisticated data science techniques.
If you prioritize hiring personnel familiar with SQL fundamentals—like navigating diverse SQL Join types to unlock data integration—you’ll build a foundation capable of supporting powerful, scalable projects. A data scientist arriving later then immediately begins adding real value instead of getting bogged down in data cleanup and integration assignments.
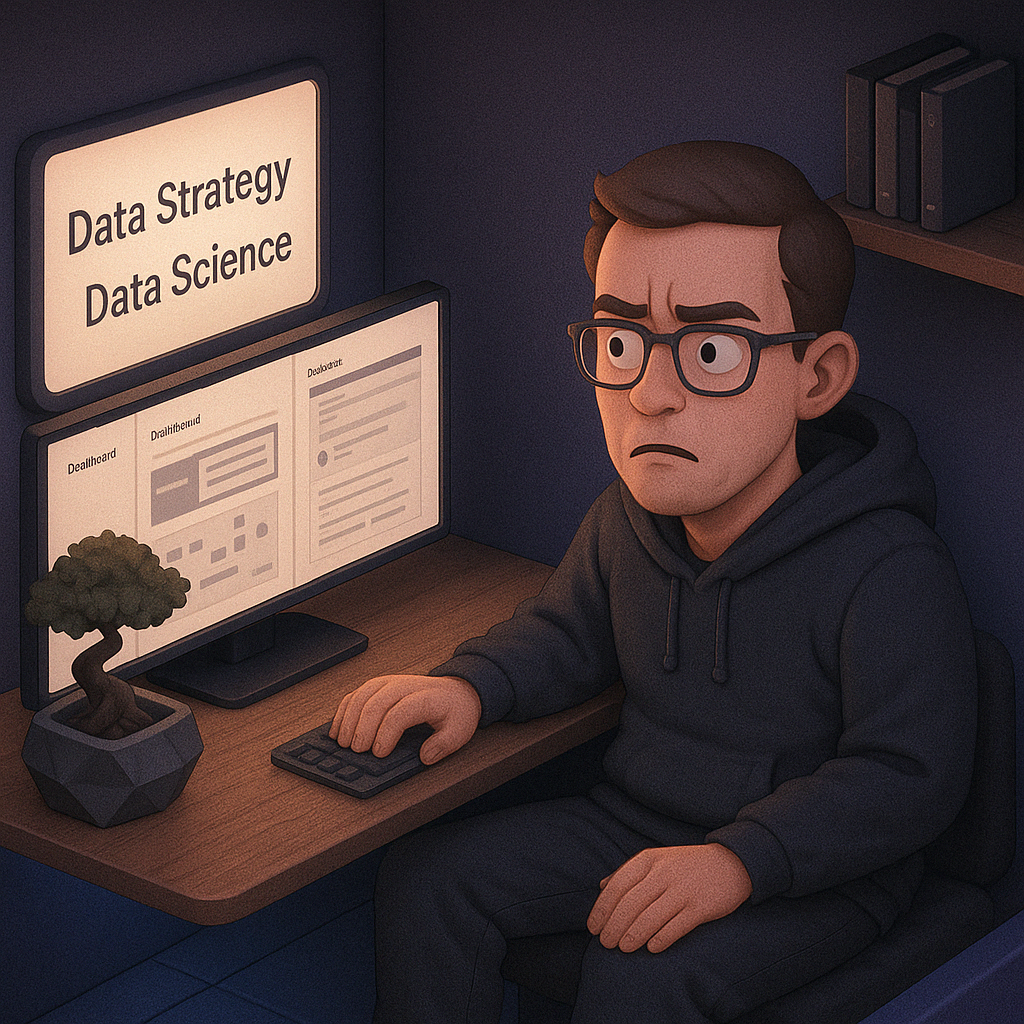
You Need to Focus on Immediate Decision-Making Needs
Before you delve into complex machine learning solutions and predictive analytics, consider the immediate decisions your business needs to make. Often, companies chasing trendy data science applications overlook more immediately beneficial analytic solutions. You might benefit immensely by starting with enhanced reporting and filtering capabilities, building interactive dashboards that enable agile, data-driven decisions over speculative predictive models that can take months to implement.
An experienced analytics analyst or analytics engineer, like those who specialize in Power BI consulting services, can quickly empower your stakeholders with interactive, dynamic visualizations. The benefits of interactive data visualization are immediate and impactful, empowering your business with real-time insight clarity and quicker decisions across all departments.
Focusing first on clear, actionable dashboards and self-service reporting platforms provides direct, measurable benefits more rapidly. Once you’re consistently using data for daily decision-making, you’ll better understand your organization’s analytic maturity. With this maturity, your data scientist hire, when it eventually happens, will already have clear mission-driven objectives aligned with your business needs.
Initial Data Strategy Should Drive Technological Investment
An effective data-driven transformation begins by outlining a clear strategy—not diving headfirst into predictive modeling. Hiring technical specialists focused on aligning your data architecture, introducing effective data governance, and identifying gaps in current business operations can profoundly influence how successful your data investments will ultimately be. It’s essential to ensure your infrastructure choices wisely set your foundation for innovation.
Data Analytics Architects and Data Engineers not only help design appropriate database solutions but they will also advise wisely regarding powerful new technologies emerging in the data sector—from streamlined data pipelines to revolutionary advancements like quantum computing. It’s worth exploring cutting-edge solutions such as quantum computing offering unparalleled processing speed. Specialists who build these foundations understand technology integration deeply and can forecast your needs accurately, equipping your business to accommodate future innovations more smoothly.
When your foundation is rock-solid, your organization is better equipped to leverage game-changing innovations like artificial intelligence and advanced machine learning. You can confidently implement plans featured in articles outlining how AI and ML technologies are transforming the data industry. Starting with strategic planning ensures your tech stack aligns with future objectives, structured intuitively for exploration by your eventual data scientist hire.
Data Science Is a Layered Approach, Not a Silver Bullet
Data scientists are undeniably valuable, but their full impact comes from building upon already-existing data capabilities, infrastructure, and cultural data literacy throughout your organization. Data science, at its core, is multi-layered: it requires well-structured data pipelines, readily available historical and real-time data, robust analytics tools, and informed stakeholders who understand how data analytics truly drive value.
Too often organizations view data scientists as one-size-fits-all solutions, expecting them to solve every analytical hurdle instantly. This unrealistic expectation inevitably leads to frustration, burnout, and suboptimal results. Sophisticated data roles should not involve endlessly cleaning up messy datasets; their responsibilities need to focus on discovering profound business insights using machine learning, developing algorithms, or even constructing advanced textual analysis tools—like this Python-based web scraping and NLP example.
Instead, gradually evolving into predictive analytics and machine learning projects allows your organization to develop key supporting structures, such as solid strategic alignment, data literacy culture, and practical analytics competencies. Thought leadership on the role of data scientists as evolving valuable team contributors can inform how best you integrate these future specialists into your team.
Domain Knowledge Matters Just as Much as Technical Skill
When making crucial business decisions, insightful and relevant domain expertise is just as important as sophisticated statistical modeling skills. Analysts and data engineers who understand the nuances of your specific business landscape—from retail to fintech to healthcare—can guide foundational analytics implementations strongly aligned with real-world challenges.
For instance, regions like Austin, Texas see industries like healthcare undergoing transformative growth through data analytics. Harnessing domain knowledge first ensures your parameters, insights, and applications focus squarely on solutions boosting tangible organizational performance. By investing initially in domain-aware analytics professionals, your teams gain contextual awareness supporting data strategy, accuracy in queries, and more actionable analytics implementation.
Data Scientists often boast excellent technical capability but may lack sufficient industry exposure and organizational context. Early in your analytics journey, domain-focused analysts and engineers will better match your organization’s current data maturity and understanding. This alignment clarifies your initial analytics goals, setting the stage for stronger integration of eventual pure data science specialties into cohesive existing processes and mature strategic frameworks.
Conclusion: Strategize for Sustainability and True Insight
Before hiring a data scientist as your first data professional, consider the long-term implications. Establishing data strategy, foundation, infrastructure, and a data-literate culture first builds a sustainable platform upon which data scientists can thrive.
Your best-first hire choices include experienced analysts, versatile data engineers, and strategic technologists. They provide the groundwork benefiting advanced analytics down the road, seamlessly integrating data science capabilities into a mature ecosystem rather than expecting magic from an unsupported individual. The final result? Insightful analytics woven deeply into organizational strategy—transforming your enterprise intelligently, confidently, and sustainably.